How organizations can leverage data and the right hybrid cloud strategy to achieve true business and delivery agility.
Sept. 29, 2021 | By Brandon Carroll
Machine learning operations (MLOps) enable smooth collaboration and communication between data science and IT teams to deploy, run and train ML models at scale. Many organizations have struggled to successfully operationalize AI and machine learning initiatives, but MLOps can be a differentiator in making a true business technology strategy impact.
How to use data to fuel MLOps for your organization
Most large enterprises are realizing what technology and eCommerce companies have known for a long time: your data is one of your largest corporate assets. Predictive analytics drive new revenue streams and open up new markets while also mitigating risk. Insurance companies have been using this model for years to establish risk parameters and establish rates, and online advertising has never been more targeted and personalized than it is today. Now, more traditional brick-and-mortar companies in industries like manufacturing and oil and gas are getting into the game.
The amount of data being collected and subsequently analyzed and modeled has increased exponentially. In a 5G world, data is coming from everywhere—not just from traditional transactional systems. Sensors and IoT devices are monitoring, tracking and sending data to all types of organizations from homes, businesses, vehicles and machines. The organizations that can successfully harness that information and use it to predict behavior can gain a differentiating competitive advantage.
The biggest challenge in adopting MLOps is the size of data sets
While organizations may recognize the potential benefits of AI and ML, many struggle to realize agility, resiliency and value from their technologies. The biggest challenge enterprises face as they attempt to adopt MLOps is the sheer magnitude of it. While organizations are collecting more data than ever, it is also less static than ever—with real-time data virtually flowing non-stop. Gone are the days of data scientists sitting in an office running models on a defined data set. ML models and corresponding algorithms must constantly change, learn and adapt to truly be successful.
Plus, like any large organizational initiative, culture can also be an inhibitor. If the business and IT are not tightly aligned, MLOps projects will not realize the benefits that outweigh the costs. MLOps is not an “IT thing” but a business value driver. Clear expectations on what the desired outcomes should be will help create the operational value streams necessary for complete alignment across all impacted internal and external parties.
Organizations can enable MLOps through the right hybrid cloud strategy
Cloud technologies enable organizations to accelerate the consumption of data products while building long-term resiliency. Hybrid cloud and multicloud environments are the prevailing architecture on which many organizations are building their business. With traditional corporate data center models, data lives in the data center, meaning, application calls must flow back and forth from end-users constantly. In a distributed hybrid cloud model, your data model is front and center in the strategy and planning. Organizations must ask themselves: What data lives in the core? What data should live in the cloud where the core applications have been migrated? What could live on the edge for lower latency and a better experience for end users?
Consider this same type of scenario for security and resiliency. In a hybrid cloud model, it may make sense to extend your security stack to the edge for more seamless access to the platforms enabling consumer services. Additionally, a hybrid cloud or multicloud strategy prevents vendor lock and allows for some level of portability as providers roll out new services or change cost models.
Consider your cloud platform more than the actual type of cloud
Savvy organizations have realized that public cloud should be an enabler to drive business value—not just a scalable, operating expense infrastructure model for operations. Internal and external consumers of cloud-based applications want real-time data, customized solutions and a pleasing customer experience. Next-gen cloud solutions should focus on repeatability, automation and optimization for that reason. The consumers of business services do not care where those services are hosted, they just want seamless access. If you focus on building scalable cloud-based platforms to deliver those services, the provider is irrelevant outside of cost considerations. For example, CaaS services like AI and MLOps can run on any model of Kubernetes platforms, whether that’s Azure AKS, AWS EKS or on-prem. And the same goes for deployment and CI/CD toolchains. If organizations focus on the platform as the enabler of driving business value, they will be successful more times than not.
About the Author
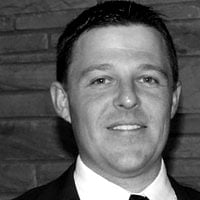
Brandon is a results-focused management professional offering 15+ years of senior-level business development and operational leadership for startup corporations and mature, rapidly expanding global operations. He possesses the recognized ability to incorporate innovative management techniques, processes and procedures to enhance business practices, increase productivity and boost revenues. He has a talent for forging strong relationships with key decision-makers, corporate executives, channel partners and employees.
Related Articles
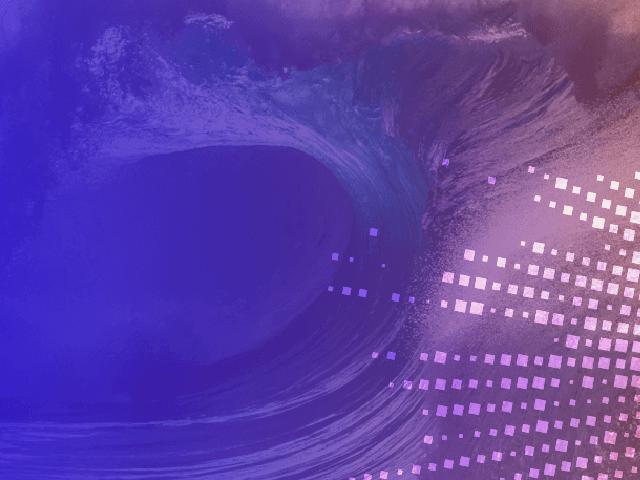
Transform your data with machine learning & AI
We believe business success is grounded in data-driven decision-making.
Explore our most recent issue of Version Next, Now where we dive deeper into data processing with AI/ML and how it delivers valuable insights.