Taking Your Data to New Depths
How AI/ML Can Help Turn the Tide
The Change Agent
Successful ML adoption requires a holistic cultural and mindset shift, wherein every corner of the organization understands how a data-driven approach will move the company forward.
Drowning in Data
Organizations are inundated with data from a myriad of sources. Data is generated from customer, vendor and client interactions, from device sensors, software and application telemetry—and the list goes on. Data is the heart of today’s digital enterprise, and having a data strategy is critical to being successful. Companies often start with technology solutions to unlock the value concealed in their data, but the humans tasked with applying that tech can quickly become overwhelmed. That’s why savvy organizations are accelerating the use of artificial intelligence and machine-learning technologies in an attempt to harness their data more effectively and create new revenue streams, improve customer experiences, augment employees’ intelligence and operate more efficiently. According to Forrester, over 50% of enterprise technology decision-makers have already implemented or are in the process of implementing AI and ML.1
Enterprises are well-aware of the potential benefits of AI and ML. New use cases continue to emerge that promise to create agility and resiliency in the organization. However, organizations are failing to realize value from AI, deep learning and other machine-learning technologies. Why is it such a struggle?
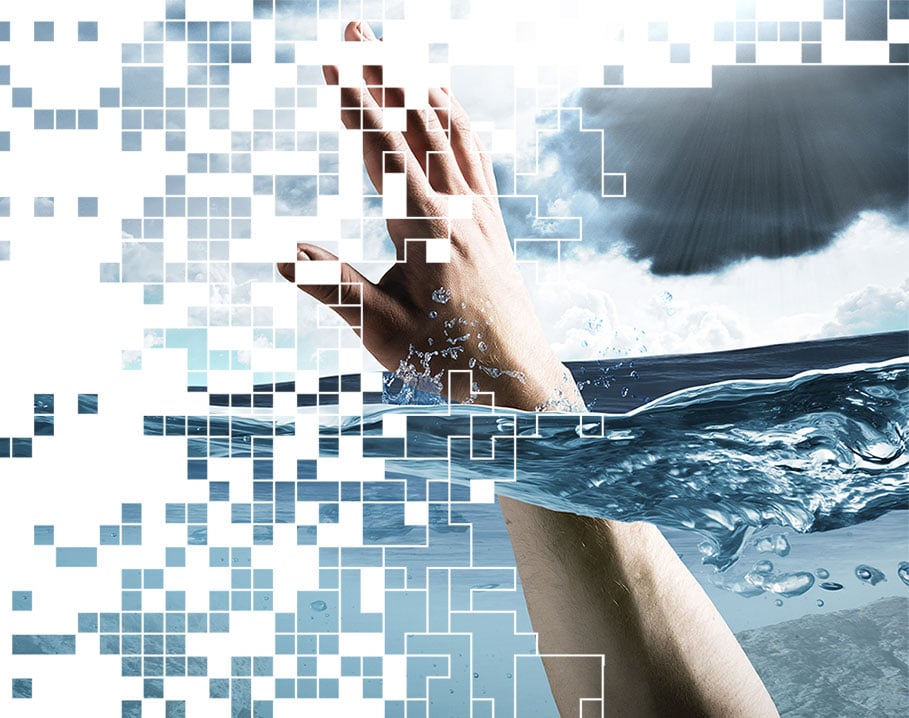
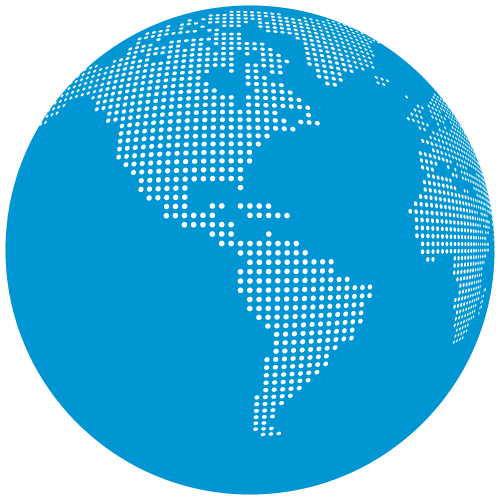
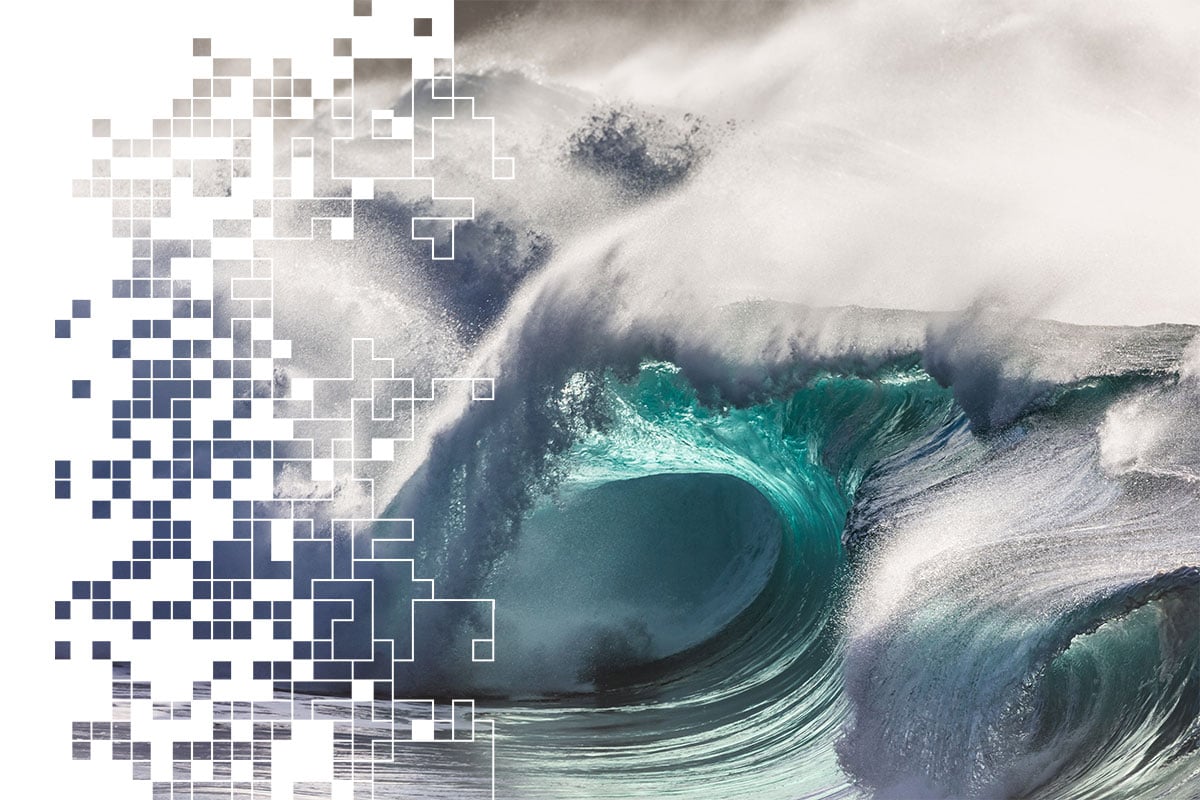
The biggest challenge is the sheer size and scope of datasets; they are massive. And in many cases, they change in real time. Until recently, most companies were dealing with manageable amounts of data and deploying a relatively small number of models in isolation. That trend has shifted as organizations embed decision automation into a wide range of applications, creating new technical challenges that come from building and deploying ML-based systems. Projects frequently stall out when attempts are made to scale up to realize the full potential of AI and ML. In fact, research from IDC indicates that around 28% of AI/ML initiatives fail.3
Company-wide adoption faces several other hurdles to success:
- Lack of senior-level buy-in: Leadership may not view AI/ML initiatives as realistic strategies or leaders have been burned by failed attempts in the past.
- Organizational silos: ML initiatives often operate in isolation from each other, making it difficult to align workflows between disparate teams, systems and datasets.
- Technical debt: ML projects include a broad array of technologies, tools and frameworks. Legacy systems and technical debt can slow transformation.
- Models are imperfect: ML models are trained on data from a snapshot in time; as data changes, the models must be frequently retrained and fine-tuned.
For organizations that have adopted a DevOps culture, many of these challenges are familiar. Similar to DevOps transformations, successful ML adoption requires a holistic cultural and mindset shift, wherein every corner of the organization understands how a data-driven approach will move the company forward. Enter machine-learning operations (MLOps). Creating an environment where this culture will thrive doesn’t happen overnight. It starts with being cloud smart. Thinking strategically about how your organization will consume cloud services will enable business agility. Then taking the right platform-based approach so your businesses can enable MLOps to realize the true value of AI and ML at scale.
Machine learning operations (MLOps) enables smooth collaboration and communication between data science and IT teams to deploy, run and train ML models at scale.
Market Perspective
AWS Global Tech Lead for MLOps Mario Bourgoin shares his thoughts about what organizations should think about as they get started with MLOps.
Getting started with MLOps
How do you define MLOps?
Mario Bourgoin (AWS): Machine-learning operations (MLOps) consists of producing, deploying, monitoring and maintaining the machine-learning (ML) components of production systems. This uses a combination of automated tooling and human intervention. It includes the following:
- Gathering, cleaning and labeling operational data.
- Creating features from the data and storing in a repository.
- Using ML methods and features to train models, either automatically or under human control.
- Deploying the models. First, deployments are in a staging environment. Then, they are in production systems. This includes the ability to roll back models to previous versions.
- Monitoring deployed models for data and model drift. This is done for input data, target concept, prediction bias, prediction explanations and business effectiveness.
- Retraining models using new data. Retraining is either on demand, on a schedule or triggered by model monitoring.
- Documenting the execution of the above steps. This includes data and model lineage. It is used in system maintenance and debugging, and to satisfy regulatory obligations.
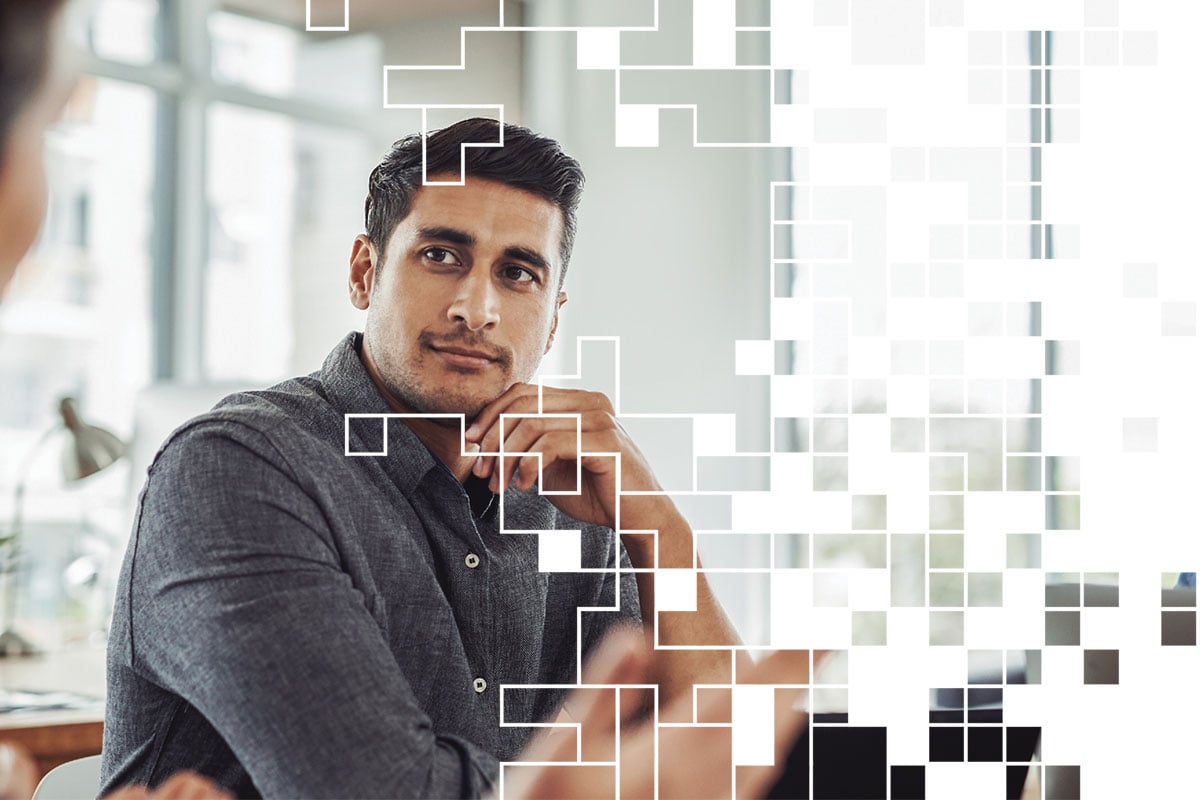
What are some of the benefits of an MLOps solution?
MB (AWS): The benefits of MLOps include:
- Greater visibility into the production of ML components and the use of ML-based systems.
- Increased reliability of the deployment of ML components and increased performance of the components themselves.
- Increased ability to scale out the number of models and scale up the complexity of models produced.
- Better focus on an organization’s goals rather than on production of ML components because of the automation of repetitive tasks.
- Alerts for human intervention for model tuning or replacement.
How should an organization get started with MLOps?
MB (AWS): They can start with adding MLOps tooling to the production of one ML component to gain experience with the process and its success factors. Then, they can commit to adding MLOps tooling to their other ML components. After that, they inventory and triage the components to prioritize their migration. And they identify each component’s sources of data, labeling methods, ML algorithms and addressed business problem. Finally, they align their ML component development process with the requirements of MLOps. This includes tracking the lineage of the data used and ML components developed.
An organization can start with adding MLOps tooling to the production of one ML component to gain experience with the process and its success factors.
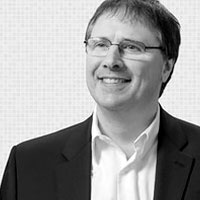
Our Perspective
TEKsystems leaders Brandon Carroll, Jay Mozo and Ramesh Vishwanathan share their points of view on how the right platform-based approach will enable data to work for the organization.
Put Data to Work for Your Organization
In an era where data is the catalyst for change, organizations are leveraging MLOps, data-driven development and AI tools to create value, generate new revenue streams and improve customer experiences. In the next evolution of the cloud journey, the focus turns to building the platforms that will enable data to work for the organization.
Data has evolved from being viewed as a supporting asset into a mission-critical component of success. A well-devised data strategy is crucial to maximize the value of enterprise data. Machine-learning technology can create considerable competitive advantage by:
- Improving visibility and uncovering more insights from data
- Improving efficiency of external and internal processes (i.e., optimizing your value stream and operations)
- Enabling a better understanding of your customers by anticipating their needs, allowing you to serve them better
- Reducing costs considerably
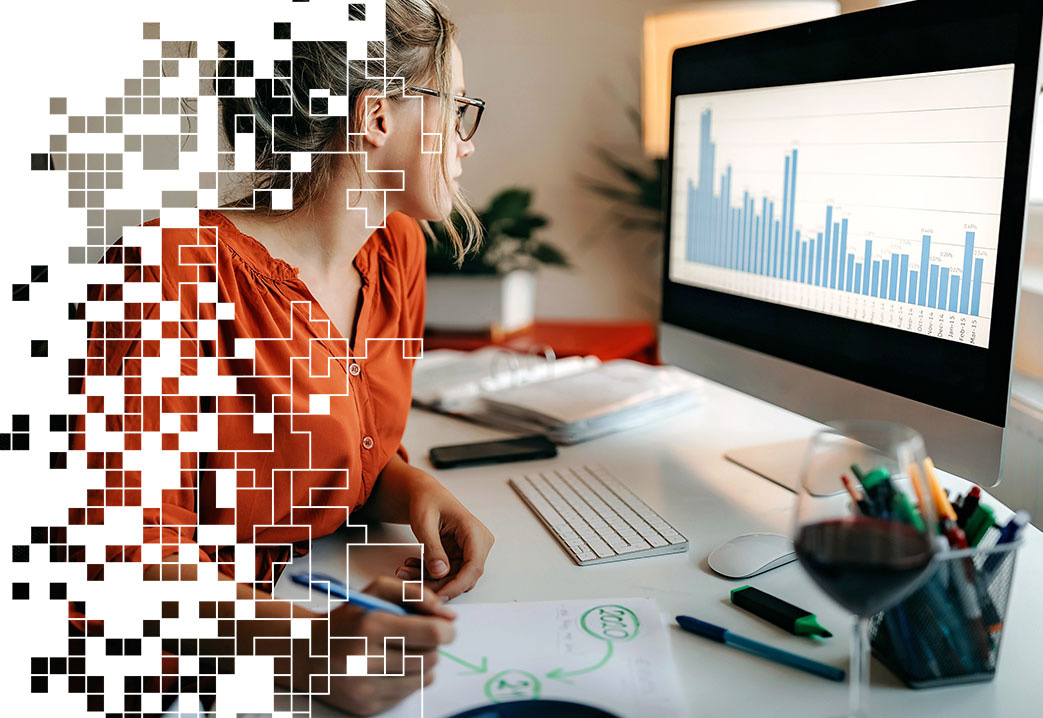
From global mining operations that deploy sensors to track maintenance schedules on massive machines to financial institutions using ML models to identify fraudulent transactions in real time, the potential applications of ML in your data strategy and value stream are nearly limitless. The consumers of your data, whether employees or customers, want the fastest and most reliable access to that data. They want data now, and they want repeatable, dependable ways to extract intelligence from it, so they can act on it.
You’re enabling MLOps through the right hybrid cloud strategy.
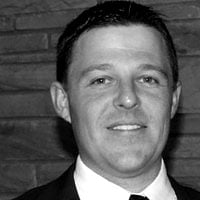
Cloud technologies enable organizations to accelerate the consumption of data products while building long-term resiliency. Hybrid cloud and multicloud environments are the prevailing architecture on which many organizations are building their business. But it’s not just about the technology—it’s more important to understand why and how to leverage the technology. In fact, starting with the technology and dismissing the need for a solid data strategy frequently stalls the project, and in some cases leads to outright failure. Businesses have been embracing the modern cloud for nearly 20 years, yet they still fail to consider several key aspects of cloud adoption.
- Cost: Buyers often completely underestimate the cost. They’re enticed by the ultimate savings potential of the cloud, but initial costs can still be high.
- Security: Data breaches are always a concern, but disjointed messaging and lack of alignment between IT teams, line of business, security teams and leadership are often the biggest barrier to adoption.
- Licensing: Many buyers are caught off guard by complicated licensing terms and how that can affect other software agreements.
- Planning: Regardless of the cloud solution, during modernization efforts you still must leverage legacy systems. Organizations often jump in and act without formalizing a strategic roadmap.
Thinking cloud smart and insight-driven enables your business to leverage multiple cloud philosophies.
Cloud Agnostic
The organization is thinking strategically with a focus on building a sustainable and automated technology foundation. You’re preparing the business to consume cloud services from anywhere.
Cloud Appropriate
Your hybrid infrastructure is flexible and allows portability as new service offerings come to market. The business has flexibility without needing to rearchitect the entire network. You have the freedom to choose the cloud services that best meet your needs and deliver the intended outcomes.
Abraham Lincoln is credited with saying, “If I had six hours to chop down a tree, I’d spend the first four hours sharpening the axe.” Whether Lincoln actually spoke these words, the point is worth considering. Approaching the cloud without a plan and, more importantly, failing to take the right strategic approach is the biggest mistake organizations make. The company frequently starts their journey by selecting a platform. They’re seduced by the features and think it will fix all their problems. In some cases, that might even seem like the right approach—until it’s too late. For instance, say marketing chooses a product because the API fully integrates with their CRM platform. Or the IT department selects a product based on the capacity for virtual servers or the product’s ability to scale containers. Both groups identified benefits that deliver value, but without a holistic approach, they’ll never capitalize on the potential of MLOps. Instead of choosing a technology based on features and benefits, think about your end customer and work backwards.
Organizations must leverage MLOps across every facet of their business. You improve the full life cycle and benefit from end-to-end value.
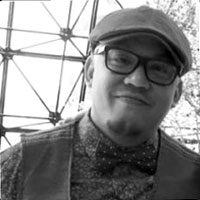
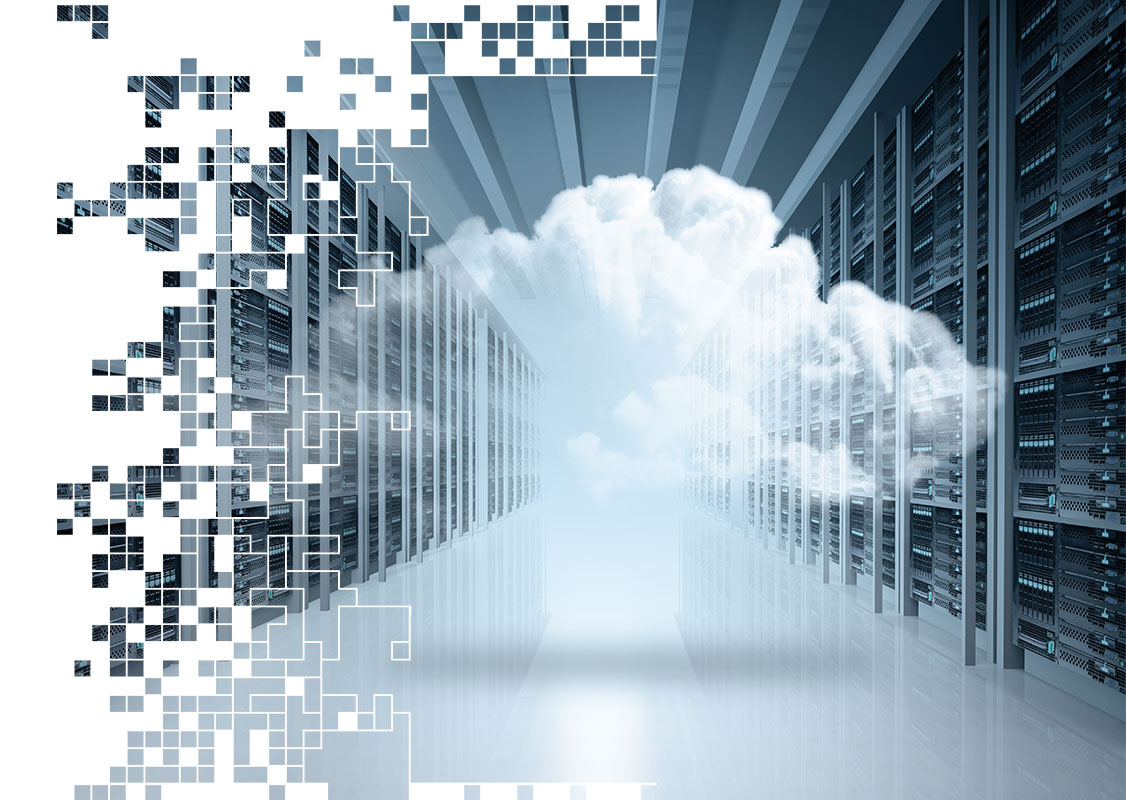
Start with the end in mind
- Start with a focus on the outcomes: What do you want to achieve or provide for your end customer?
- Next, think about the processes: What do you need to enable the desired outcomes?
- Lastly, determine the platform: What platforms or products will enable our process?
Starting with a plan and building a roadmap will help you construct operational value streams and enablement runways that will serve as the foundation for achieving true business and delivery agility.
The biggest challenge in most organizations isn’t about lack of activity on the data science team. It’s that they fail to embed the analysis into a true outcome-driven process.
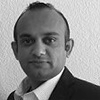
Meet Our Contributors
Sources
- A Guide To Navigating Our AI Technology Research Portfolio, Forrester
- The World Will Store 200 Zettabytes Of Data By 2025, Cybercrime Magazine
- AI Strategies View 2020: Executive Summary, IDC