Master the Momentum of Technology
Think of TEKsystems Global Services as your growth solution. Your guide through disruption and into the future. Partner with us for our proven expertise in technical strategy, design, execution and operations.
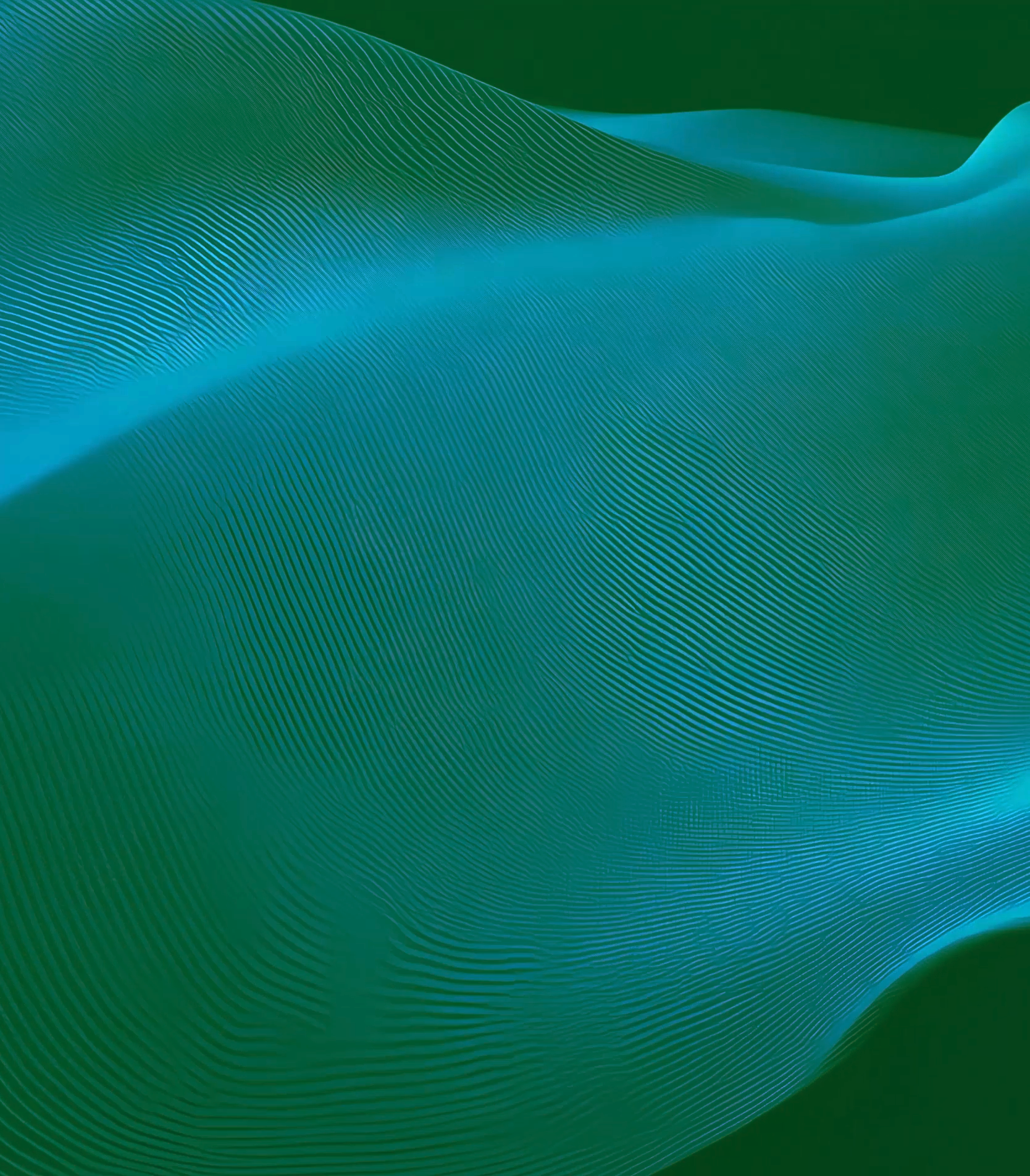
What We Do
Your business is our priority. As your partner in IT and business services, we’ll deliver on your goals from Day 1.
Taking Flight With AWS
A leading global airline, our customer wanted to reimagine the baggage experience for passengers. By shifting to Amazon Web Services (AWS), they greatly improved baggage claim platform speed and scalability.

Advancing Business Solutions with Autonomous AI Agents
In this issue we explore how agentic AI is redefining what’s possible across the enterprise—from transforming customer experiences to accelerating innovation and operational efficiency. Up against mounting pressure to do more with less, agentic AI offers autonomous systems that can interpret context, make decisions and act—without constant human oversight.
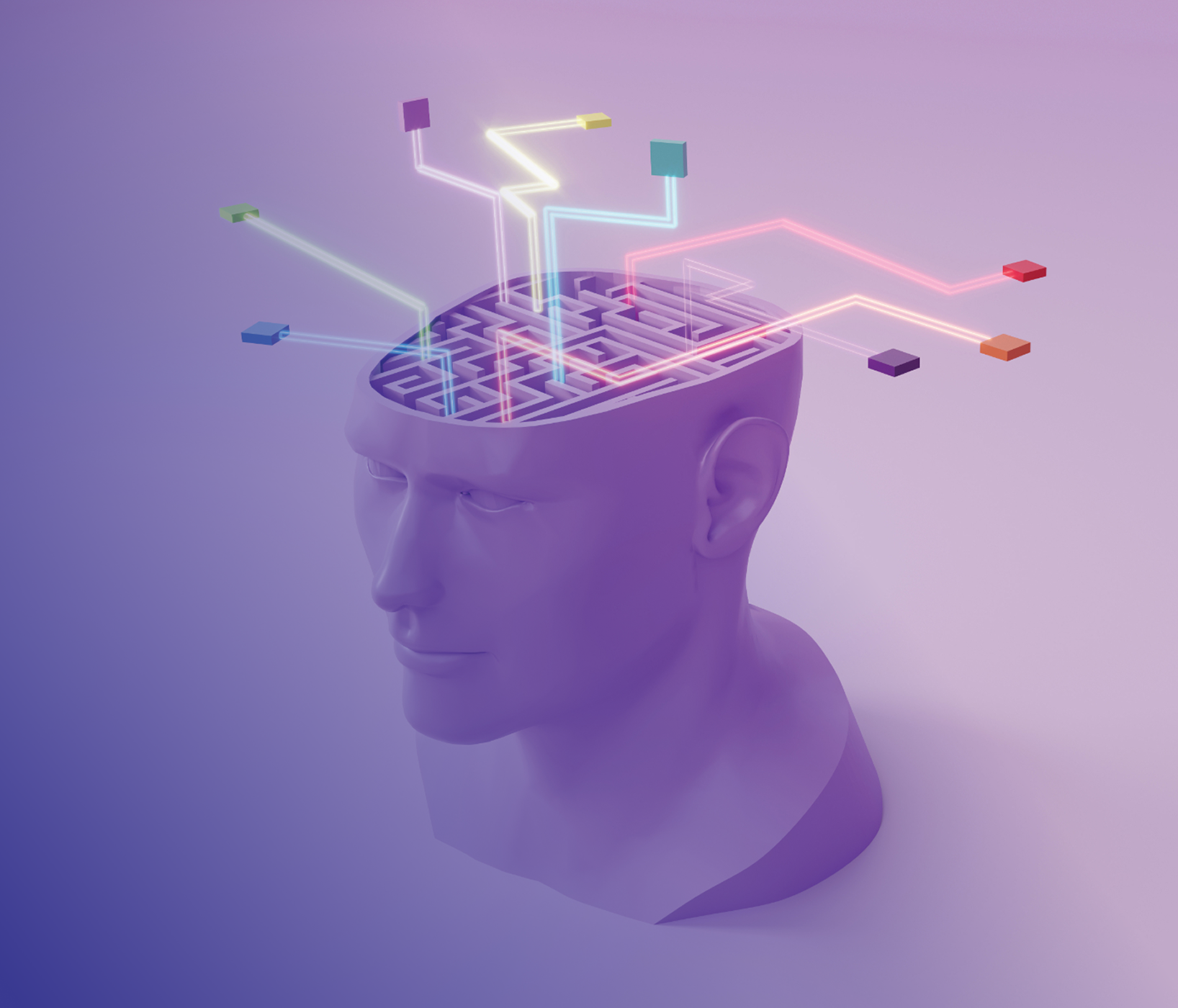
Let's Master the Momentum
TEKsystems Global Services specializes in technology services and consulting. We develop and execute cloud, data and customer experience solutions that help businesses master the momentum of technology.
With deep expertise in strategy, design and operations, we partner with leading technology providers to drive growth for customers.
Our Approach
How We Do It
Banking on Digital Strategy To Enhance Customer Experience
TEKsystems Global Services helps a large bank holding company implement a next-generation digital experience, requiring a comprehensive end-to-end data and testing strategy.
Our Company at a Glance
We Lead Customers to Success on a Global Scale


Customer retention
NPS scores for services delivered
Higher customer satisfaction rate than the competition’s
Thinking Forward
Let’s Own Change, Together
Explore how TEKsystems is working side by side with customers to create real-life impacts on organizations and the people they serve.