Paperless Precision
Unleashing NLP in Business Management
The Change Agent
In an era where efficiency and accuracy are paramount, businesses are constantly seeking ways to streamline operations and reduce manual workloads. Increase productivity and revolutionize modern business operations with natural language processing (NLP) and generative AI.
A New Era in Document Management
Imagine a world where mountains of paperwork are handled effortlessly, where contracts, reports and emails are meticulously organized and understood in mere seconds. In this world of advanced document management and generative AI, businesses operate with unprecedented efficiency thanks to the seamless integration of cutting-edge technology. This digital transformation is not the stuff of science fiction, but a present-day reality made possible by the advancements in natural language processing (NLP).
NLP, a subfield of artificial intelligence, focuses on the interaction between computers and human language. By leveraging sophisticated algorithms and vast amounts of data, NLP enables machines to comprehend, interpret and generate human language in a way that is meaningful and useful.
From automating customer service responses to analyzing vast troves of legal documents, natural language processing use cases, enhanced by generative AI, are revolutionizing business operations across various industries, making tasks that once seemed insurmountable now manageable with the click of a button.
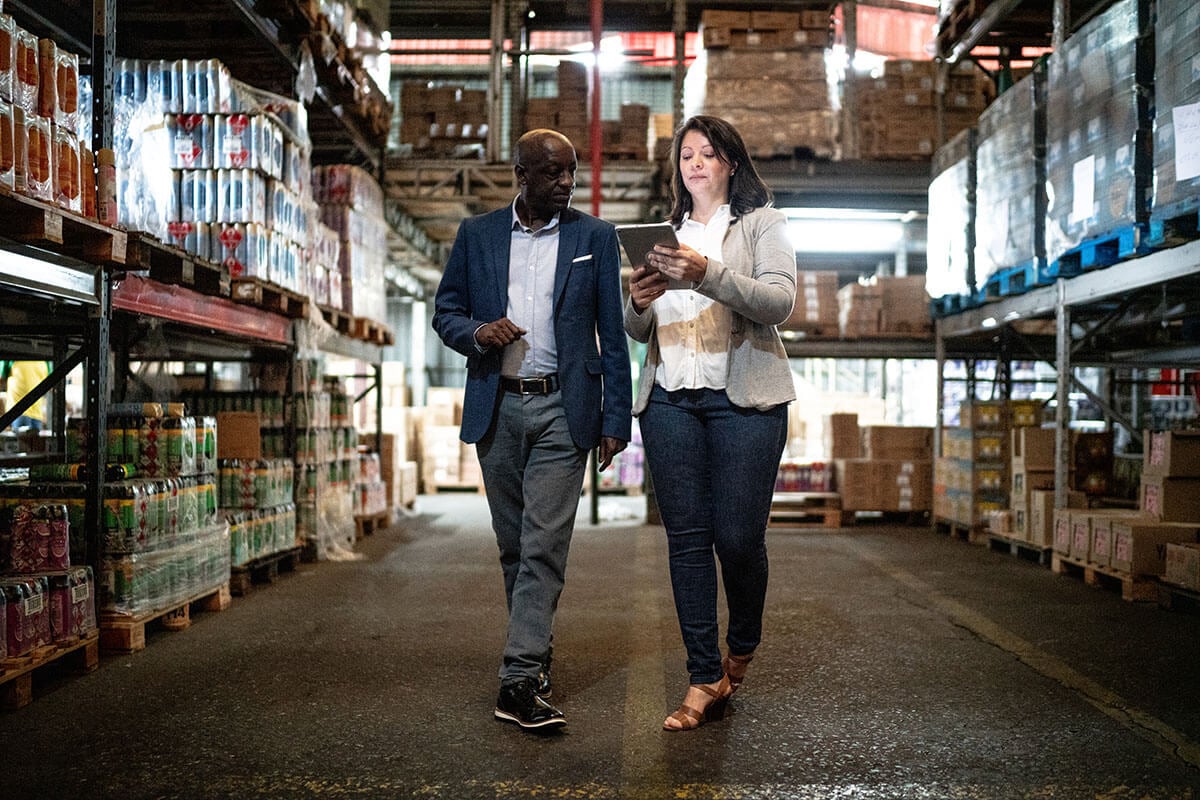

Organizations must think about how to combine the best of both worlds, providing specific information about guidelines and expectations for a particular domain or industry rather than just generic responses. This is where NLP, fueled by Gen AI, becomes a differentiator.
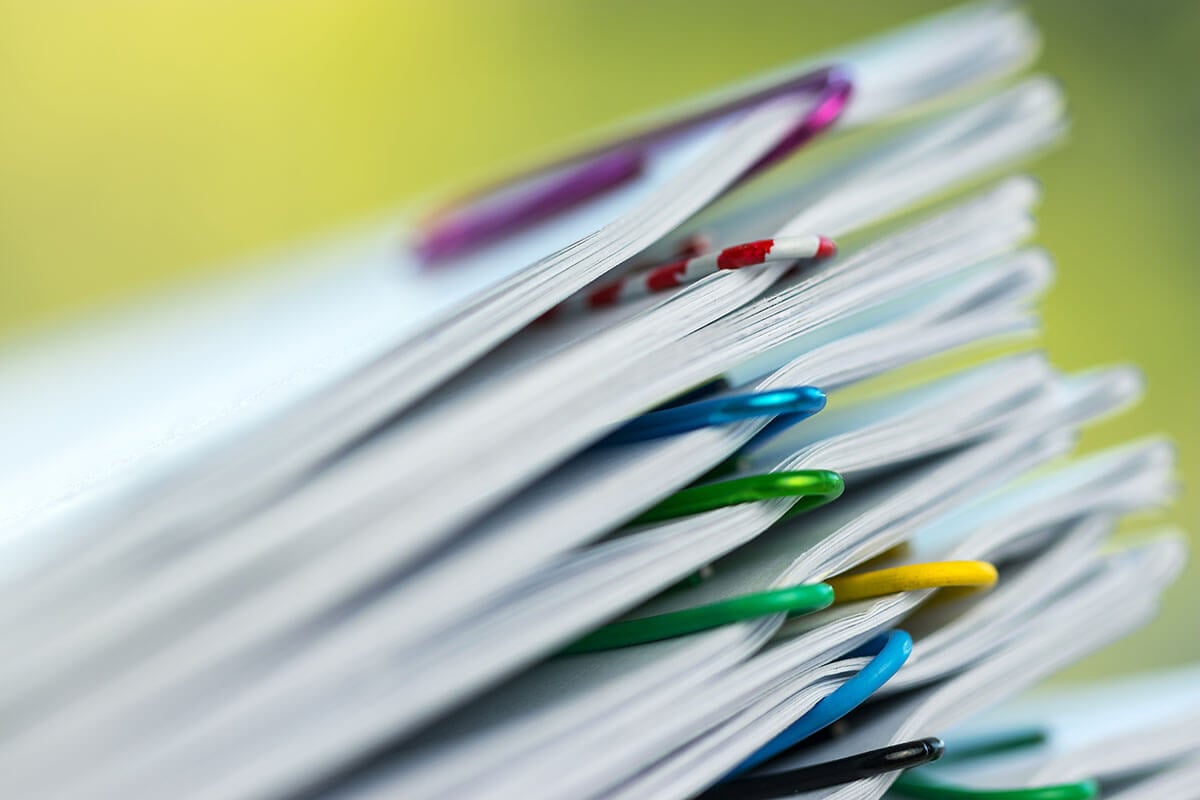
As we delve deeper into the NLP applications and capabilities, we uncover the true potential of this transformative technology. Traditionally, document management used to involve time-consuming manual processes that were prone to human error. Employees had to sort, categorize and file documents by hand, often leading to inefficiencies and bottlenecks. Searching for specific information within these documents was akin to finding a needle in a haystack, and the physical storage of paper documents required considerable space and resources.
In contrast, modern Intelligent Document Processing (IDP) solutions, powered by NLP and enhanced by generative AI, have redefined document management for businesses. IDP automates the extraction, classification and validation of information from a variety of document types, both structured and unstructured. These systems can swiftly process invoices, contracts and forms, accurately pulling relevant data and integrating it into digital workflows.
The shift from traditional methods to IDP solutions exemplifies the profound impact of technology on modern business operations. By adopting IDP, organizations can streamline their workflows, reduce operational costs and allocate human resources to more strategic tasks, ultimately driving productivity and innovation.
The global NLP market is projected to increase from $36.42 billion in 2024 to $156.80 billion by 2030, reflecting a compound annual growth rate (CAGR) of 27.5%.1
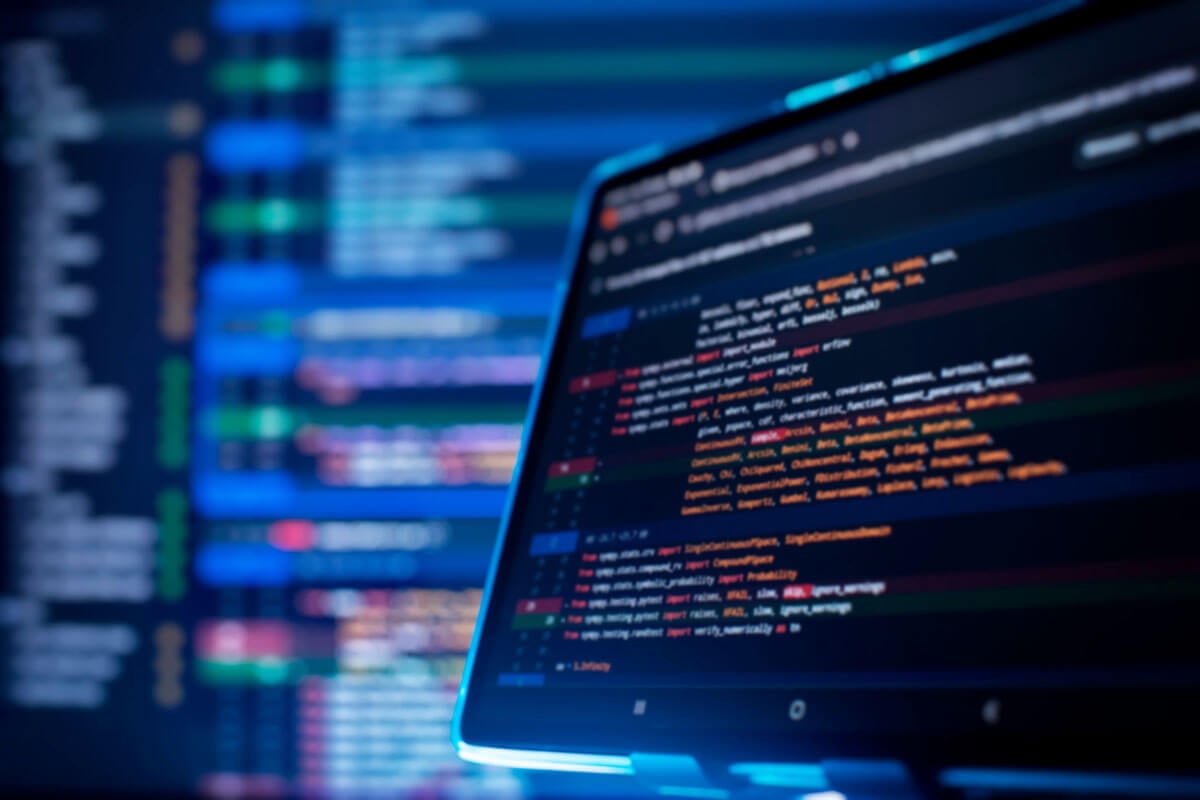
How Does NLP Work?
NLP works by combining computational linguistics with machine learning and deep learning techniques. It is a transformative technology that enhances the ability of machines to understand and interact with human language. Its integration into Intelligent Document Processing significantly improves efficiency and accuracy in handling large volumes of documents, enabling businesses to automate and streamline various processes.
The process typically involves several key steps:
A Brief History of NLP
1950s
Alan Turing proposes the Turing Test to measure a machine’s ability to exhibit intelligent behavior.
1960s-’70s
Development begins for early rule-based systems and the first chatbots like ELIZA.
1980s-’90s
Statistical methods and the development of algorithms like hidden Markov models (HMMs) for part-of-speech tagging are introduced.
2000s
Machine learning techniques and the use of large corpora for training models are on the rise.
2010s
Deep learning models emerge, such as recurrent neural networks (RNNs) and transformers, leading to significant advancements in NLP tasks. Specific NLP functions in Intelligent Document Processing leverage NLP to automate the extraction, classification and analysis of information from various types of documents.
2019 and Beyond
Large language models develop.
To effectively reap the benefits of natural language processing, organizations need a robust data strategy that ensures the availability, quality and relevance of data.
Market Perspective
Amit V. Singh, AWS global head of GTM, generative AI and machine learning partnerships, shares his point of view on Intelligent Document Processing (IDP), highlighting IDP’s AI-driven efficiency in automating document tasks, AWS’s role in enhancing IDP with machine learning, and the diverse industries benefiting from these advanced solutions.
AI-Driven Efficiency in Document Processing: Insights From AWS
Can you explain what IDP is and how it differs from traditional document processing methods?
Amit V. Singh (AWS): Intelligent Document Processing (IDP) is a widely recognized industry term used across various sectors to accelerate paper-based or digital business processes and minimize costs by leveraging AI for automatic extraction, classification and analysis of information with high precision.
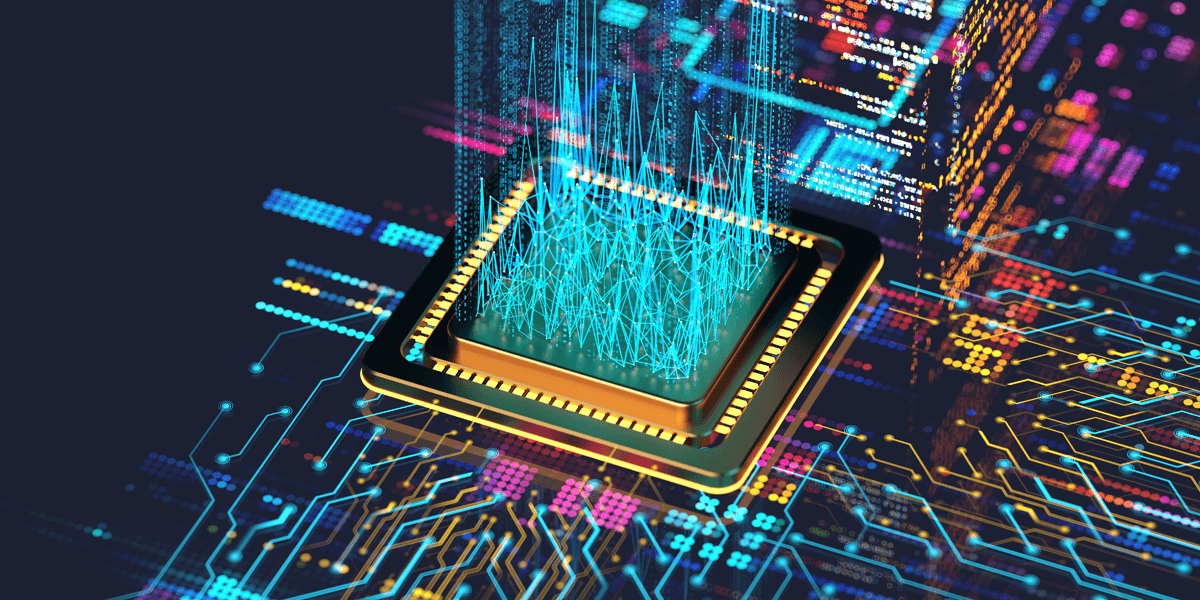
Amit V. SinghGlobal Head of GTM, AWSIntelligent Document Processing (IDP) is a crucial technology used across various industry sectors to streamline the processing of a large volume of documents, often reaching millions per year.
How does AWS leverage machine learning and artificial intelligence to enhance IDP solutions?
Amit V. Singh (AWS): IDP utilizes a combination of AWS services, including Amazon Textract, Amazon Comprehend, Amazon Comprehend Medical and Amazon Bedrock. Amazon IDP is powered by a combination of pretrained AWS AI services APIs, providing industry-leading accuracy for extracting data from unstructured and semi-structured documents such as forms, notes, invoices, receipts and tables.
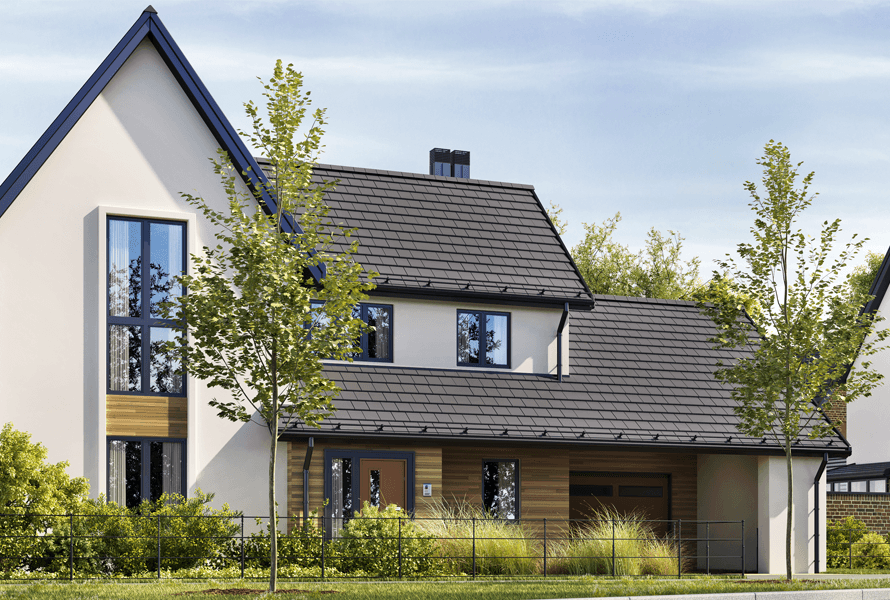
What are some common use cases and industries that benefit the most from IDP solutions provided by AWS?
Amit V. Singh (AWS): IDP is a crucial technology used across various industry sectors to streamline the processing of a large volume of documents, often reaching millions per year. This technology finds significant application in sectors such as financial services, insurance, the public sector, healthcare, life sciences, legal and manufacturing industries. AWS IDP places particular emphasis on key focus industries, including financial services (i.e., mortgage and insurance sectors), as well as the public sector and healthcare. Some specific use cases include [the following]:
- A leading mortgage lender with over 4 million lifetime customers offers a wide range of mortgage options. They reduced document processing time from hours to minutes using AWS IDP.
- A financial services-oriented technology company for mortgage and home equity lending and services achieved an accuracy of more than 90% leveraging AWS IDP.
- A major health insurance company, which provides health coverage for over 47 million members through affiliated health plans, utilized AWS IDP to automate 90% of their document classification for claims processing.
Our Perspective
By integrating NLP into IDP solutions, organizations achieve higher accuracy, efficiency and scalability in document processing, leading to significant cost savings, improved compliance and better decision-making.
NLP and IDP Synergies
IDP uses advanced technologies to automate data extraction, interpretation and processing from various document types. NLP significantly enhances IDP by improving text recognition, understanding, data extraction, document classification, search and retrieval, risk management, workflow automation, and user experience.
NLP enhances Optical Character Recognition (OCR) for better text recognition and correction, especially in noisy or low-quality scanned documents. Beyond text extraction, NLP provides semantic understanding, allowing systems to grasp content meaning. Techniques like named entity recognition (NER) identify and classify entities within documents.
Automated data extraction, powered by NLP, accurately pulls key data points from documents like invoices, contracts, forms and emails, reducing manual effort and errors. NLP also automates document classification into predefined categories, improving organization and routing within workflows. Clustering and tagging enhance document management by grouping similar documents and adding relevant keywords for easier search and retrieval.
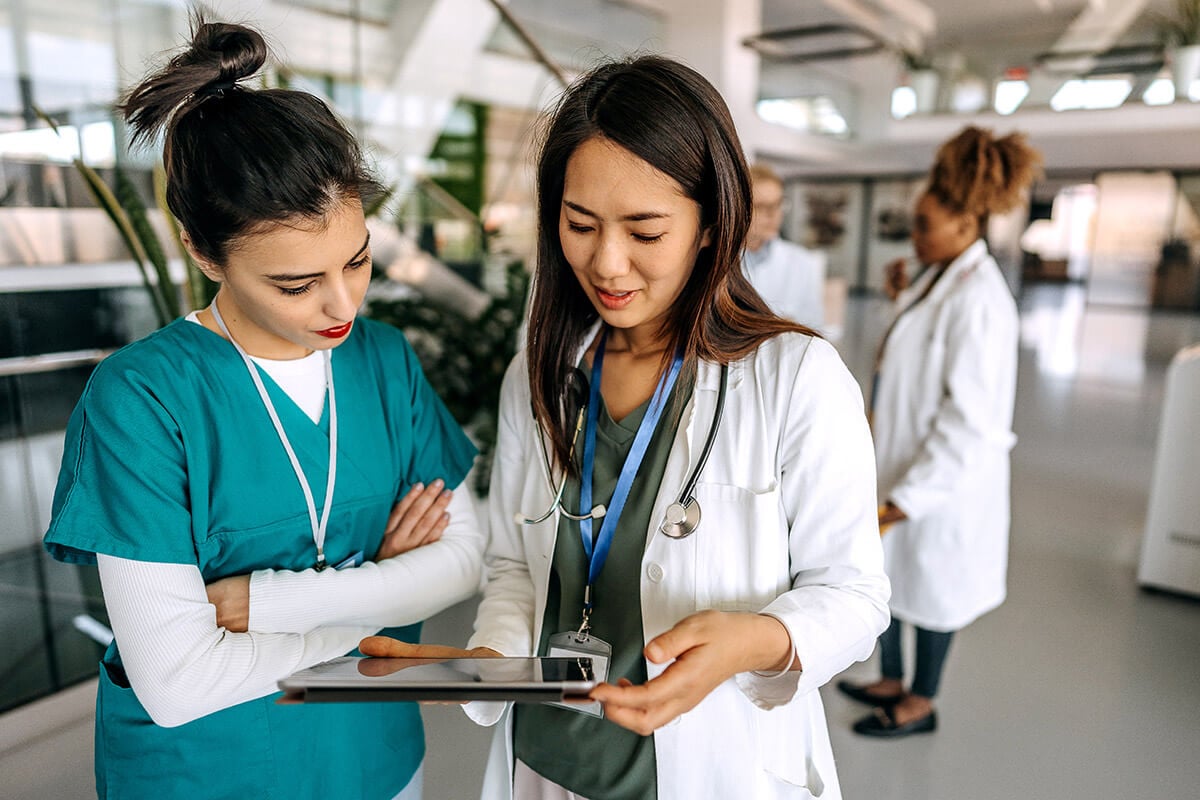

What changed since generative AI came into play is that generative AI has been trained on how humans communicate, and that training was not done like the old deterministic training methods, giving NLP responses more humanist qualities.
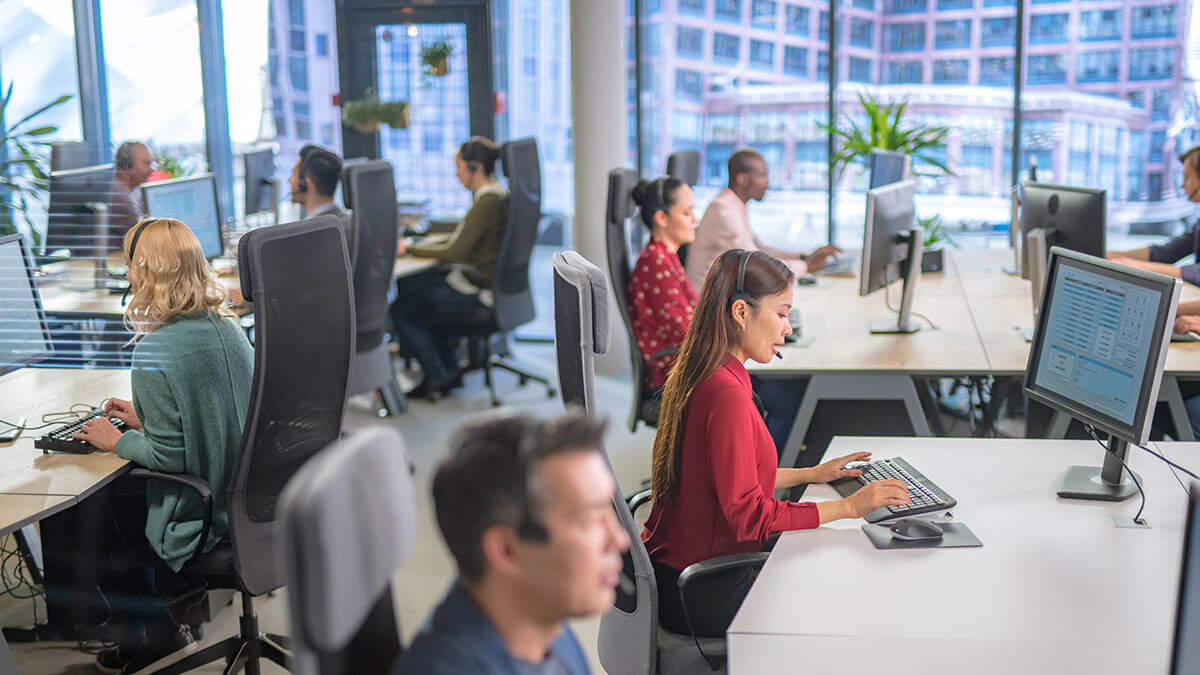
Industries most in-demand for NLP are financial services and healthcare.
Enhanced search capabilities, driven by contextual and semantic search, allow users to perform more intuitive and accurate searches, particularly useful in domains like legal, medical and technical documentation. NLP improves data normalization and standardization, ensuring consistent formatting and enriching extracted data by linking related information.
NLP enhances compliance and risk management by identifying and extracting information required for compliance reporting, auditing documents for standards, and flagging noncompliant content. It automates end-to-end document processing workflows, integrating with systems like ERP, CRM or RPA platforms, reducing manual intervention and increasing efficiency. Intelligent routing capabilities direct processed documents based on content and context.
Lastly, NLP enhances user experience by providing intuitive interfaces for interacting with document processing systems through natural language commands and queries, making systems more user-friendly and accessible.
The convergence of NLP with IDP uses advanced technologies to automate the extraction, interpretation and processing of data from various document types across an organization. Generative AI enhances these capabilities further by interpreting complex documents such as doctors notes and technical diagrams, providing summaries and insights that older AI models couldn’t achieve.
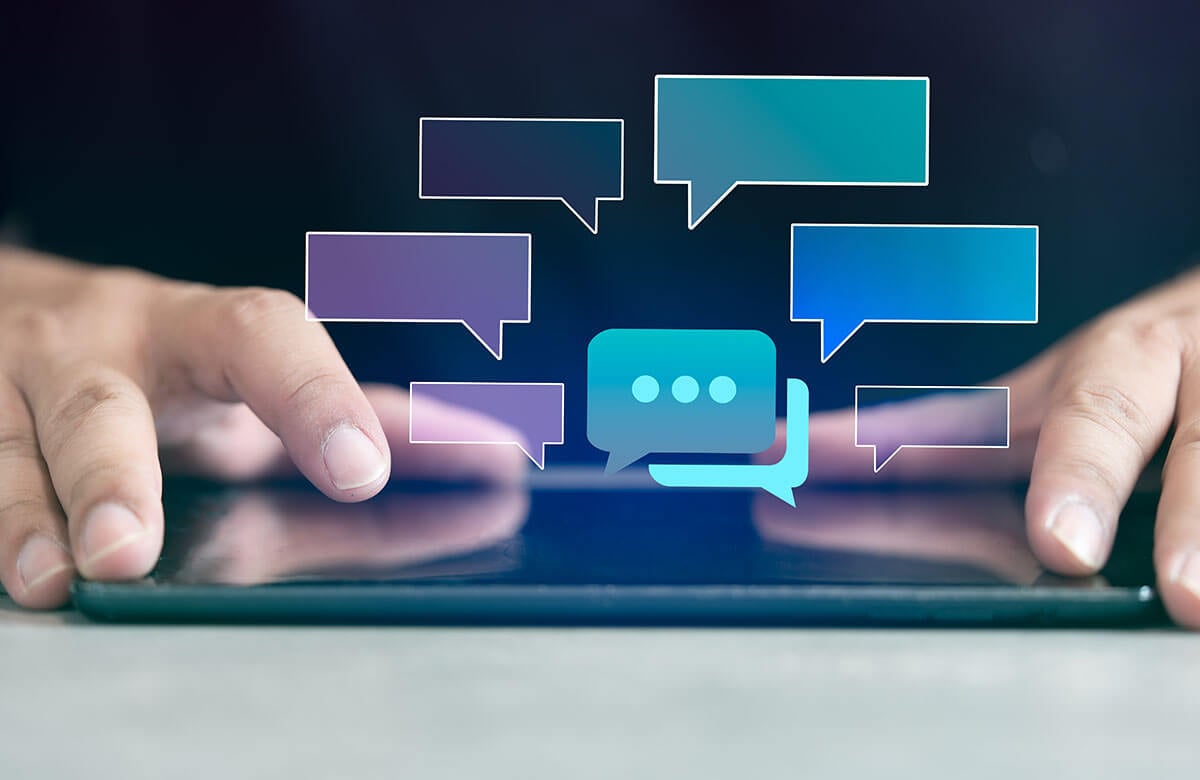
Challenges of NLP-Driven IDP
NLP faces challenges like language differences, data training, ambiguities, misspellings, bias and phrases with multiple meanings. These challenges impact its effectiveness and efficiency.
Languages vary in syntax, grammar and semantics, making it difficult for NLP systems to perform well across multiple languages. Training NLP models requires large volumes of high-quality, annotated data, which is resource-intensive and time-consuming. The quality of training data directly impacts model performance, with inaccuracies or biases leading to poor results.
Developing NLP systems involves complex processes requiring expertise and computational resources. Rapid advancements in NLP mean keeping up with the latest techniques can be challenging. Natural language is inherently ambiguous, with words and sentences often having multiple interpretations, requiring sophisticated contextual understanding.
Misspellings and typographical errors in real-world text data challenge NLP models’ accuracy. Bias in NLP models, learned from training data, can lead to unfair outcomes, requiring careful selection and preprocessing of training data. Many words and phrases have multiple meanings depending on context, necessitating large amounts of contextual data and sophisticated modeling techniques for effective disambiguation.
Addressing these challenges requires ongoing research, advanced techniques and comprehensive strategies to ensure NLP systems are accurate, fair and robust.
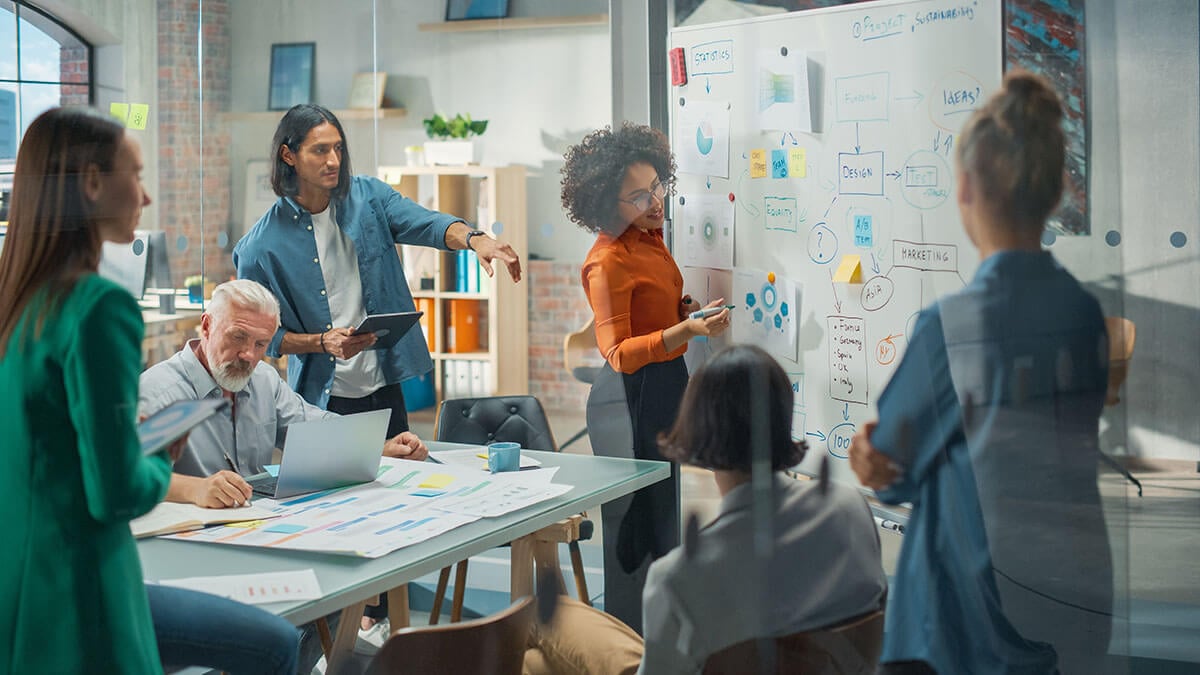
Benefits of NLP-Driven IDP
IDP—a Gen AI solution—will be a game changer.
NLP offers numerous benefits in business operations, customer interactions and data analysis. One primary benefit is enhanced customer service. NLP-powered chatbots and virtual assistants provide 24/7 support, handling routine queries and freeing human agents for complex issues, resulting in faster response times and higher customer satisfaction. Sentiment analysis tools monitor customer feedback in real time, allowing prompt issue resolution.
NLP improves efficiency and automation by handling repetitive tasks like data entry, document processing and information extraction, minimizing errors and increasing operational efficiency. In finance, it automates information extraction from financial documents, speeding up loan approvals and compliance checks. In the legal field, NLP streamlines contract analysis and legal research.
NLP enhances data analysis by extracting insights from unstructured text data, informing strategic decisions, improving marketing campaigns and providing deeper customer understanding. It helps organizations maintain a competitive edge by monitoring and analyzing competitor activities and market trends.
NLP contributes to better decision-making and knowledge management by improving search result accuracy, aiding quick information retrieval and enhancing productivity. Intelligent document processing solutions categorize and tag documents, making retrieval efficient. This is beneficial in fields like healthcare, improving access to relevant medical literature and patient records.
Despite challenges, implementing generative AI and NLP in IDP solutions offers significant benefits, including higher accuracy, efficiency, and scalability, leading to cost savings, improved compliance, and better decision-making.