Data Untangled
How organizations are harnessing the power of data to drive strategic, profitable and sustainable growth
The Change Agent
The technology landscape is constantly in flux. As technology evolves, so does the customer—and their expectations are a moving target. Behind the scenes, data is quietly being collected across the enterprise, waiting to be translated into meaningful business intelligence.
Empowered by Data
Every organization has a not-so-secret weapon: data. Incredibly valuable information generated from every aspect of the enterprise. From research and development, to the supply chain, sales performance, human resources, marketing, operations as well as customer service and behavioral insights. Every activity in every facet of the business provides more data. Periphery data outside of businesses’ control, such as social media, syndicated or other public data, is critical, too.
The volume of available data is almost incomprehensible. In fact, there are an estimated 2.5 quintillion bytes of data being created every day,1 and the world’s collective data will grow from 33 zettabytes (ZB) in 2018 to 175 ZB by 2025, according to IDC.2
For organizations planning to stick around, harnessing the power of data is a business imperative because of the opportunity it holds: competitive differentiation. When armed with the knowledge and capabilities to digest data and extrapolate meaningful insights, organizations are poised to make informed and strategic decisions about all areas of the business. In turn, these data-driven decisions can help save time and money, create new revenue streams and exceed customer expectations.
A data strategy is a set of choices and decisions that together, chart a high-level course of action to achieve high-level goals. 4
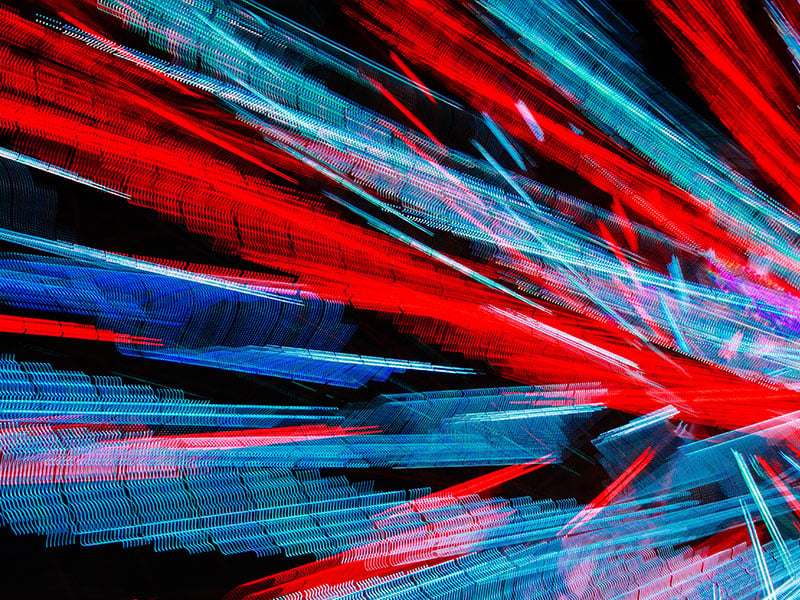
Unfortunately, many organizations struggle with using data to their advantage. The reasons include:
- Overcustomized Technology: Legacy ERP systems can be overengineered to fit an enterprise’s precise needs. This level of customization becomes burdensome to maintain and scale over time.
- Costly New Technology: Replacing the old with the new, on-premise implementations can be a massive investment.
- Misguided Technology Selection: Best-in-class enterprise applications and systems demand the integration of multiple technologies; mistakes can be made in this important process, hampering connections. On the other hand, by going only with standardized platforms, organizations may sacrifice desired features and tools.
- Lack of Talent: Organizations may be eager to hire a data scientist to help them navigate their data. However, there is a shortage of data scientists equating to more than 150,000 unfilled jobs3 in the U.S.
- Managing Expectations: If they do want to hire a data expert, organizations need to recognize the shortage of professionals with these niche skills and the high cost of employing them. Most organizations admit needing an innovator who can deliver transformational analytics solutions; many do not allocate the budget required to attract that caliber of talent.
Success with data starts with a data strategy.
A data strategy helps organizations map the business objectives, needs and priorities they’re trying to solve for with their data. It ensures future technology decisions and business activities are sound and aligned with enterprise goals. Establishing a data strategy—and reviewing it regularly—not only paves the way to informed decision-making and successful business outcomes, but it also positions the organization to competitively thrive.
In fact, research proves that productivity and profitability improve when an organization is empowered by data. Businesses that strategically leverage their data see an estimated $430 billion in productivity benefits compared to their less data-friendly peers, according to the International Institute for Analytics.5 And organizations that incorporate big data and analytics into their operations realize productivity and profitability rates that are as high as 6% greater than the competition.6 Cost and productivity efficiencies directly help the bottom line. Data puts them one step ahead and ready to apply technologies such as AI and machine learning.
Market Perspective
Working where data meets compelling business intelligence, Amazon Web Services (AWS) leader Andy Bhaduri and Tableau leader Charles Schaefer share their points of view on data strategy, challenges and best practices for optimizing the power of enterprise data.
Data with Purpose
What are the biggest challenges you see organizations struggling with when it comes to getting the most out of their data?
Andy Bhaduri (AWS): I believe the biggest challenge for organizations today is visualizing and executing on a data transformation journey. If you ask any business or functional leader in an organization, they will have a strong grasp of their business or function and can list several ways in which they can improve their revenue, profitability or operations using data-driven insights. Similarly, organizations also have some grasp of where data already exists or sources of new data to serve the business. Traversing the path from data source to data-driven business impact is the most challenging. It not only needs deep technical capabilities at the data management layer, but also deep business understanding to model and derive insights and predictions from that data.
Charles Schaefer (Tableau): Some of the most common challenges I see are:
- Too difficult to access data: In many cases, the governance models and policies that organizations employ to keep their data secure and their environments performant restrict access to the people who are in the best position to use the insights derived from it to improve decision-making and business outcomes.
- Data silos and inconsistencies: Because it is too difficult to access data—and/or the “single source of truth”—data silos often emerge and operate outside of the purview of IT. This not only leads to data inconsistencies across silos, but also creates inefficiencies across the organization due to data replication, unnecessary movement of data and a greater risk of data misuse/abuse.
- Data literacy gaps: Even when business users get access to the data they need, they often lack the skills and overall data literacy to prepare and analyze data to find and validate insights. In many organizations, the act of preparing and analyzing data is left to a small group of experts or power users, which limits scale and overall organizational impact.
- Culture change is difficult: Many organizations talk about their desire to be data driven and rely on analytic processes to drive their decision-making, but few organizations have achieved this vision across the enterprise. A significant, and often difficult, culture shift is required to move from making decisions based on instinct to relying on insights derived from data analysis.
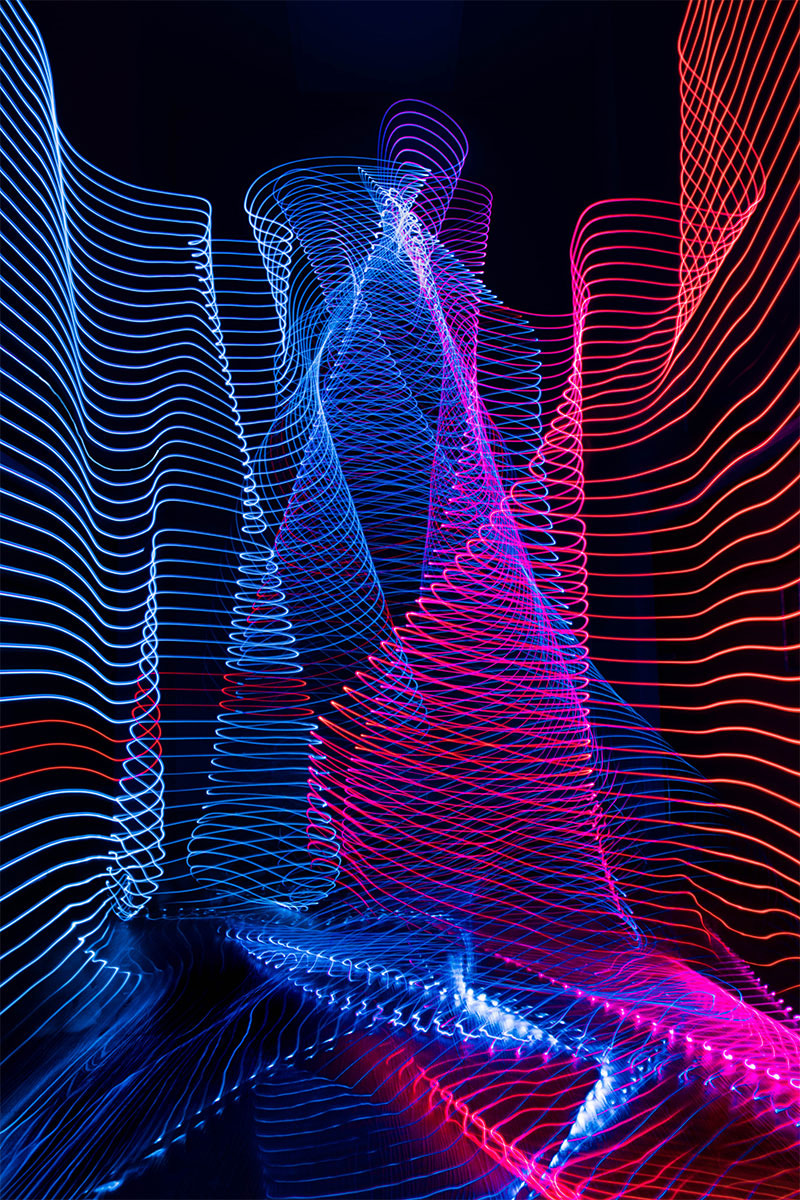
What is the value and purpose of a data strategy?
CS (Tableau): Organizations have a lot of options when considering how to collect and store data, how to make it available to decision makers, and how to govern it to assure sensitive information is not put at risk. The decision processes related to these options can be made more efficient with clear policies—avoiding constant touchpoints whenever a business group adopts a new system, for example. Organizations reduce risk by determining who can access and analyze data, and those with a clear data strategy can closely align data with strategic business initiatives, generating true business value with data.
AB (AWS): Data strategy cannot be thought of or created in isolation but is inextricably linked to your business strategy. If your business strategy is to become more customer centric, you need to identify specific points of impact (for example, delivering the right offer to the right customer at the right time) and then work backwards in deciding what data you need to deliver that impact. Once you have the start and end points, you need to create the data strategy to delivering that impact. Data strategy can be for an individual initiative, a bunch of initiatives or company-wide, but they all follow the same principle—what pathway do we create for our data to deliver the desired business impact?
How does a data strategy relate to the overall goals and priorities of the business?
AB (AWS): As mentioned, data strategy cannot exist in isolation. It is akin to saying that you have a car but nowhere to drive. Data strategy, like HR strategy or sales strategy, is an integral component of delivering a business goal. It is not without reason that more and more organizations have a chief data officer role similar to a CFO, CMO or CHRO role.
CS (Tableau): Data is closely tied to decision-making. As more organizations make all their processes digital, they can trace the decisions they make to ideas that started with insights found in data. Even organizations without any strategy around data understand that it’s important to make decisions based on facts, but a data strategy can represent “how” business goals and priorities will be achieved by outlining expectations for how those decisions are made.
When do you recommend creating a data strategy?
AB (AWS): I think it would be erroneous to think of data strategy as something that you create because data is the topic du jour. Data strategy should be an integral part of your business strategy. You create business strategy on day one—or day zero—of your business, and at that point you need to create a data strategy. There is a reason why you see businesses like Uber, Airbnb, Amazon and others as disrupters. These organizations think of data strategy as a core part of their business strategy. For existing business, if you are still waiting to create your data strategy, you might already be late because your competitors are creating theirs.
CS (Tableau): In a lot of ways, it’s an overarching goal, not an endpoint. If an organization avoids working with data until they have a perfectly defined data strategy that encompasses all possible inevitabilities, they’ll be stuck forever as technology changes and data piles up exponentially. But a lot of organizations have never created a data strategy, and they have a hard time knowing what information they can trust because they don’t know how any of their insights were collected. It’s something you invest in over and over, adapting as your business changes.
It would be erroneous to think of data strategy as something that you create because data is the topic du jour. Data strategy should be an integral part of your business strategy.
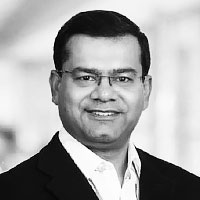
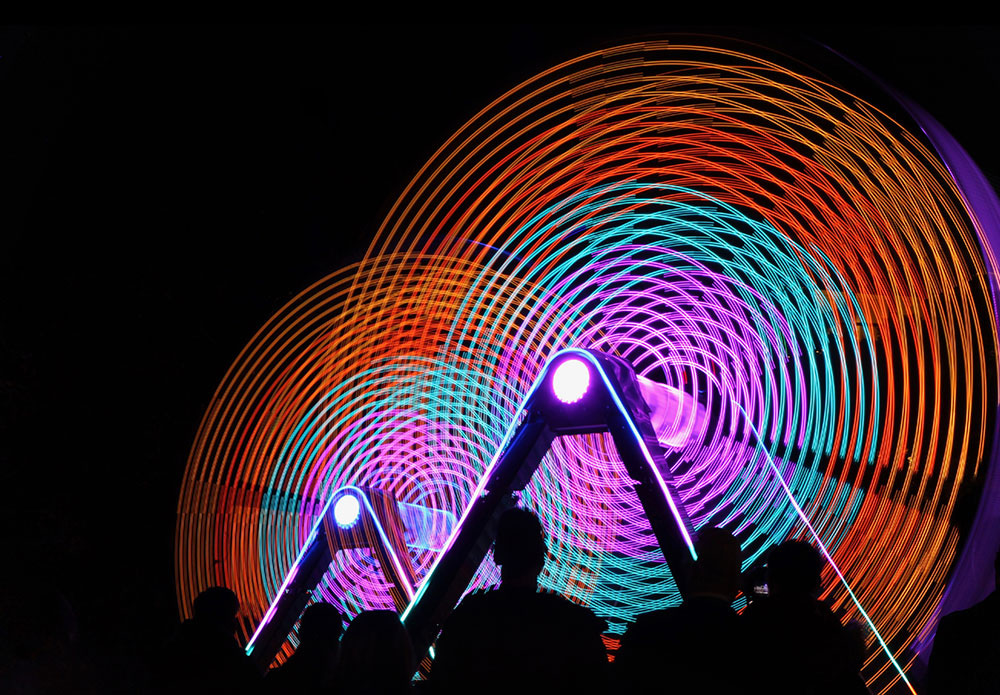
What does a data strategy look like?
CS (Tableau): It starts with a few high-level beliefs: What do we want people to do with data? How do we want people to access it? How much technical skill do we expect our people to have? These beliefs must be both aspirational but also accept the reality and expertise of your team. Then, there are processes that stem from those beliefs that help define how data will be managed to meet those goals. This is where you start to get into the technical solutions (e.g., metadata management, ETL, when and where to define schemas around data, etc.). But remember, a well-defined data strategy should be founded in business outcomes, with the technical processes serving to help optimize every decision that an organization makes.
AB (AWS): A data strategy, just like any other strategy, should serve an overarching vision or goal that it is set out to achieve. Going back to the customer centricity example, a good business goal might be revenue from coupon usage (right coupon at the right time to the right person). The data strategy should deliver on this business goal. It should include a data management plan, guiding principles for data assets (i.e., when to retire, when to use, who gets access, etc.), data governance plan and overall success metrics. In this case, the data strategy serves only one business case, but in other scenarios, a data strategy might serve a collection of business cases or an entire company.
Who owns the data strategy?
CS (Tableau): There really needs to be C-level accountability for an organization’s data strategy, and many that have recognized this have created CDO, CAO or CDAO roles, often accountable directly to the CEO. With the focus on business outcomes and alignment to an organization’s overall goals and strategic objectives, these roles are ideally on the business side even though input is needed from technical groups to make it successful. Ultimately, everyone in the organization “owns” the success of a data strategy because it takes the buy-in and participation from everyone to truly make an impact enterprise-wide.
How often should organizations review their data strategy?
CS (Tableau): A data strategy should be a living and breathing thing, revisited on a regular cadence. When something changes—a new data source is onboarded, for example, or a line of business is created—this should trigger an assessment of how the process is influenced by the data strategy and whether the strategy should be amended. If key metrics and KPIs deviate from what is expected, a change in data strategy might be evaluated to understand why organizational metrics aren’t being met or are failing to drive the expected outcomes. And technology is evolving so fast that sometimes a new innovation inspires a forward-thinking company to re-evaluate its data strategy to become more efficient or save money.
What common pitfalls do you see organizations struggle with in their data strategy?
AB (AWS): I see two kinds of pitfalls as organizations try to define and execute their data strategy. The first one is being “too ambitious.” Many organizations tend to think of data and associated benefits as the panacea of all their woes. Data can indeed transform businesses and lead to big benefits, but it takes a lot of effort and time. Organizations tend to underestimate this effort and draw up ambitious plans only to feel let down when they do not achieve their lofty goals or achieve smaller victories. The second pitfall is at the other end of the spectrum. Organizations tend to think of their data strategy as a technology issue rather than a business issue. They build data lakes and then struggle to deliver business impact because that was not part of the strategy to begin with. Organizations feeling dissatisfied with benefits from their data lake strategy is a well-documented issue in the industry.
CS (Tableau): Organizations can get bogged down in procedural, data management processes while losing sight of the strategic goals a data strategy is ultimately created to serve. This often happens when companies fail to adapt. By putting the operational elements first, businesses end up limiting the insights they can gather; whereas if they focus on how to get the facts they need to impact their business, they can refine a data strategy over time to actually drive business value. This isn’t to say that governance is unimportant; it’s crucial. But it has to govern the right things to fuel self-service analytics.
Technology is evolving so fast that sometimes a new innovation inspires a forward-thinking company to re-evaluate its data strategy to become more efficient and save money.
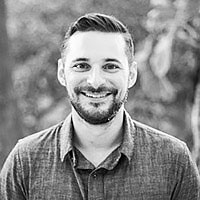
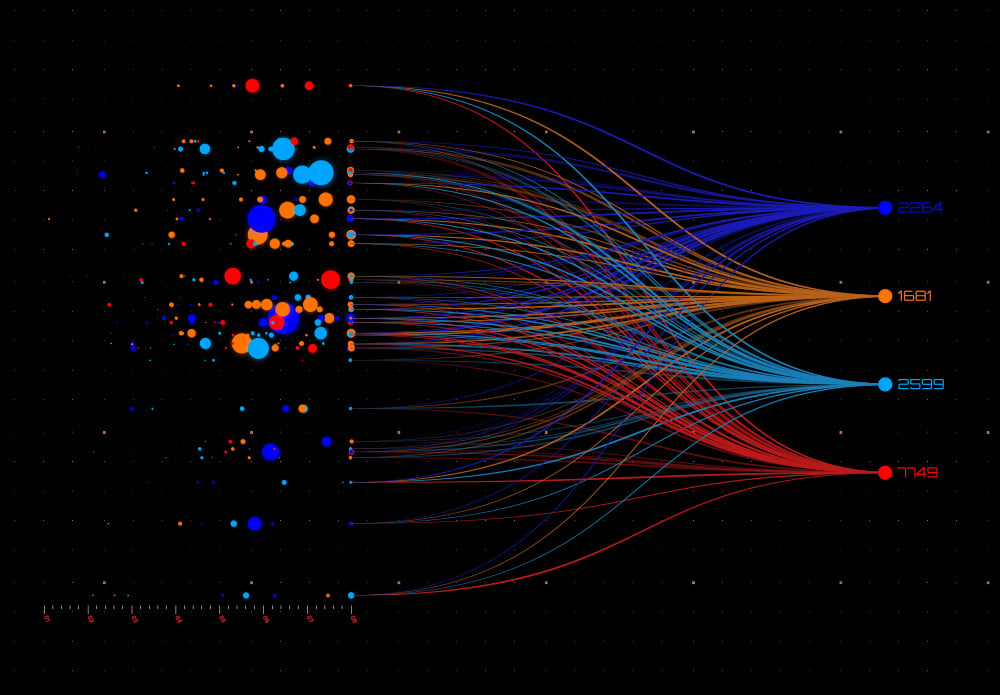
How do organizations measure the effectiveness of their data strategy?
CS (Tableau): First, look at the extent to which business outcomes have been improved by the data strategy. Are people getting the information they need to make crucial business decisions, and are those decisions being made with impactful results? But there’s something else that organizations should be encouraged to measure: the adoption of the policies created by a data strategy. If business users can’t get the information they need, they usually find another way. That often means going outside of policy to uncover, store or export data in ways that put information at risk. Or they resort to simply making decisions based on gut feel. Looking at software usage, for example, can show how often people are actually using the processes and tools you provide them.
AB (AWS): Measurement of the data strategy should be tied closely to the measurement of business goals of which that data strategy is serving. In the earlier example, if the vision is to become more customer centric and the goal is to achieve more coupon-driven revenue, then your data strategy goals should include both business and technical metrics tied to this goal—redemption rates, model effectiveness, number and frequency of delivering coupons, size and cost of data used, etc. Only when you tie these together can you measure and say that data is creating a meaningful impact on your business.
How do you recommend organizations future-proof their data technology investments?
AB (AWS): There are five core elements of future-proofing any technology investment, including those in the data and analytics space.
- Organizations should make technology investments that make them more agile with the ability to develop and deploy quickly and react to market and competition.
- Technology investments should also offer elasticity so that organizations right-size their infrastructure and save costs while serving market needs whenever required.
- Organizations should also think whether their data platform could enhance their ability to innovate. Does it lower the cost of experimentation? Does it democratize experimentation or concentrate it in the hands of a few highly trained people?
- With organizations doing business across the globe, they also need a data platform that can be deployed easily, securely and in compliance with regions across the world.
- Finally, a large part of future-proofing an investment is predicting costs into the future. Escalating costs are a major issue with many organizations when they get locked into their technology choices. They need to look at pricing patterns over the last several years to decide if their costs will drop or increase in the future because of their data technology decisions.
CS (Tableau): It’s critical to revisit assumptions made during the formulation of the data strategy on a periodic basis and ensure that there is flexibility and adaptability built into the strategy that will allow it to easily flex as things evolve. My recommendation is to have a steering committee that forms and reviews the data strategy, with cross-functional participation. IT members should hear how business users find insights and use the data at their disposal, and line-of-business leaders should be encouraged to stay up to date on what’s possible. When this communication breaks down, processes and technology around data grow stale without either team realizing it. When they work together, technology investments are connected closely to the needs of the business.
Our Perspective
A well-informed, well-defined data strategy is your future-state blueprint. “Most companies know they need data, but they don’t know they need a data strategy. When they want to extract value out of the data, that’s where the rubber meets the road,” says David Spires, TEKsystems’ executive director of applications services.
To be successful, a company’s data strategy needs to align with the broader goals of the business. Not only should the strategy be actionable but also comprehensive, considering the entire enterprise and not just one department.
Jennifer Kling, director of enterprise applications, data analytics and insights at TEKsystems, adds, “It’s very important to have a strategy around what you want to accomplish from an enterprise applications and analytics standpoint because it’s going to help you create a clear path for what business objectives you want to accomplish and how you’re going to get there. This is ultimately going to save you time and money.”
Success with Data
With the right data strategy in place, organizations set themselves up for success in one of three ways:
- Creating efficiencies that drive increased time and cost savings
- Identifying new or adjacent market opportunities that generate new revenue streams
- Strengthening relationships and loyalty through improved customer experiences
David SpiresApplications Services at TEKsystemsCompanies often design for perfection. But they need to be agile enough to cope and shift with the landscape.
Mapping a Data Strategy
So how does a data strategy take shape?
To begin, an organization should map out these necessary steps:
- Identify top data priorities for the whole enterprise and clarify intentions for practical data usage
- Establish a hypothesis, desired business outcomes and use cases (e.g., chatbot, natural language processing, machine learning)
- Define the type of data needed, identify data sources and plan the approach to data collection, ingestion, management, storage, analysis and governance
Throughout data strategy development, collaboration across the organization is vital. IT, the chief data officer and all lines of business (e.g., marketing, finance, HR, sales and supply chain) need to work together. Collaboration ensures there is consistency and quality with the data, eliminates redundant data and aligns the business to a single source of truth.
Before going too far, businesses can establish a proof of concept, demonstrating that solutions work before scaling. Regular gut checks and quality assurance tell you if you’re on the right track or if you need to revisit your strategy. “Companies often design for perfection. But they need to be agile enough to cope and shift with the landscape,” Spires adds.
Common Pitfalls
“The biggest mistake companies make is settling on technology-based decisions without first having a strategy in place. They don’t make the right business decisions because they are constrained by the wrong technology.” — Jennifer Kling, Data Analytics & Insights at TEKsystems
Avoid these frequent missteps when developing a data strategy:
- No common denominator: There needs to be a leader, like a chief data officer, who can identify and resolve competing priorities within the organization.
- Technology vs. business-based decisions: Technology selection should be a component of executing the strategy rather than what drives the development of a strategy.
- Hiring for the sake of hiring: Before hiring a data expert, be clear on the role this person will play.
Key Considerations
According to TEKsystems Senior Practice Director of Data Analytics and Insights Ram Palaniappan, there are several factors that must be taken into consideration when establishing a data strategy:
- Business strategy: Data is a competitive advantage only when aligned to the business strategy as it evolves.
- Security: Proper privacy and security measures are essential to maintain integrity and trust.
- Data democratization: Gatekeepers create bottlenecks. A healthy strategy enables access and consumption of data across an organization.
- Empowerment: Enable employees to take advantage of insights through self-service solutions.
- Monetization: Identify opportunities through which data can enhance or extend products and services.
TEKsystems’ Tips
“When thinking about how important data is for companies, to me it’s very similar to the pulse of a human.” — Ram Palaniappan, Data Analytics & Insights at TEKsystems
TEKsystems recommends these steps for data strategy success:
- Identify use cases: Determine the end goal you’re trying to reach.
- Achieve quick wins: Don’t try to boil the ocean; each quick win is a step in the right direction. You must understand what your use cases are. “Being able to knock out those use cases and see some quick wins justifies the amount of time and effort behind analytics initiatives and having a strategy in place,” Kling says.
- Solicit feedback: Look for common data uses across lines of business to avoid redundancy.
- Engage a strategic partner: Work with a full-stack partner that understands your data needs and goals across the enterprise. Make sure the partner can help craft the most effective strategy, technology selection and proof of concept.
- Govern data: Data is an enterprise-wide asset. Establish policies and mechanisms to control and protect it.
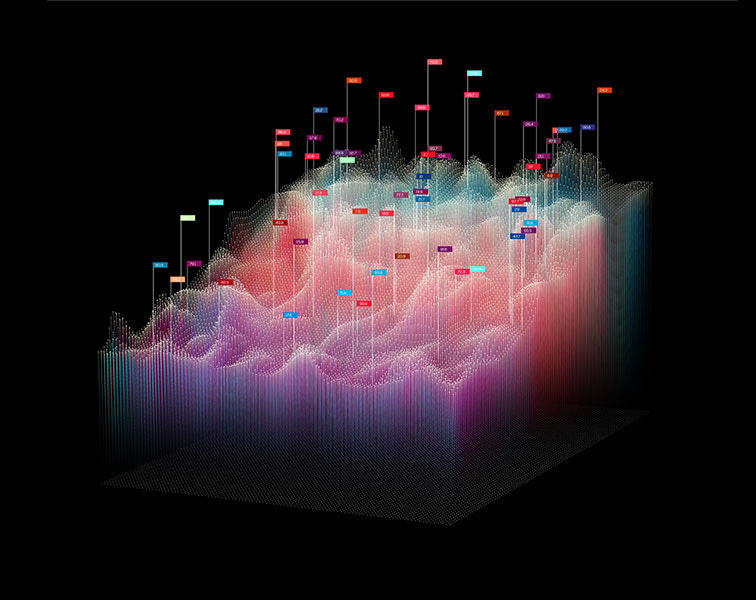
Data is a powerful resource. But extracting that data for business intelligence can be a daunting task. The organizations that recognize there are no shortcuts are one step closer to realizing its value and using it to drive their business.
Real-World Application: Netflix
Netflix is a true innovator when it comes to owning data and leveraging it for a competitive advantage. As a pioneer in digital content streaming, the company demonstrates the importance of owning change, being agile and adapting with the evolving technology landscape, says Ram Palaniappan. “Netflix quickly moved from the mail-in DVD model to exclusively streaming,” he says. “How did they find out that was what the customer wanted? The data points provided validation.”
The streaming service provider is nearing 150 million paid subscribers. In April 2019 Netflix announced it earned a record-high quarterly paid net adds—growing by 9.6 million subscribers in a single quarter.7 Strategic use of data has kept Netflix on this trajectory.
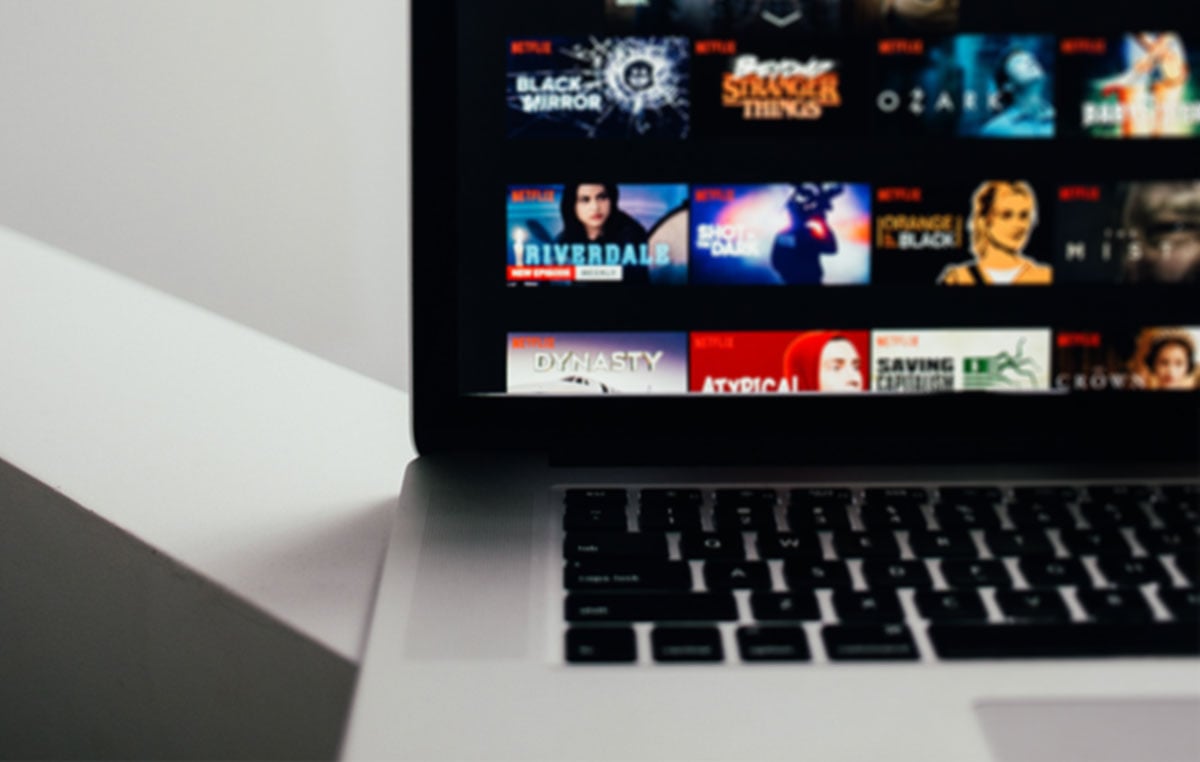
Ram PalaniappanData Analytics & Insights at TEKsystemsHow did they find out that was what the customer wanted? The data points provided validation.
Data not only offers the opportunity for cost and productivity savings and new revenue streams, but it also enhances personalized experiences that delight Netflix customers. These experiences keep them engaged and watching. Based on preferences and previous behaviors, Netflix can understand their customers in a unique way, anticipate their needs and provide recommendations. They monitor patterns such as search terms, the day of the week and the time of day content is watched, and the type of content that performs well and maintains heavy viewership. Netflix can even see at which point viewers start to decline or drop off during a program and over the long term.
This information can be used to inform multimillion-dollar decisions, such as the selection of licensed programming like Friends, and invest in new original content like Stranger Things.
Netflix’s ability to pivot based on data insights makes the company stand out in a time when competition among streaming providers is intensifying and new entrants keep penetrating the marketplace.
TEKsystems Data Portfolio
We believe business success is grounded in data-driven decision-making.
300+ customers, including 20% of the F100
Delivering expertise in cloud analytics, AI and machine learning, data warehouse and master data management, data governance and quality, big data management and IoT.
Intellectual property to accelerate delivery
800K+ lines of code of TEKsystems IP leveraged covering 55+ accelerators across AI, conversational platform, data engineering, data visualization and cloud analytics.
In good company
Transformational technologies demand equally transformative partnerships. TEKsystems is proud to deliver data insight solutions across the full spectrum of leading platforms to drive data as organizations’ competitive advantage.
The views and opinions expressed in this publication are those of the authors and do not necessarily reflect the views of TEKsystems, Inc. or its related entities.
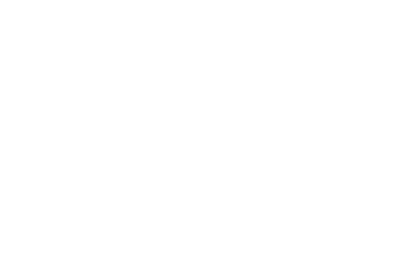
Listen Now
Don’t miss an interview with Jennifer Kling and Ram Palaniappan on The Agile World podcast. Host, author and industry expert Greg Kihlström asks Jennifer and Ram about all things enterprise applications and analytics.
Listen to the podcast on your favorite streaming service
Apple Podcast | Spotify | Google | Stitcher
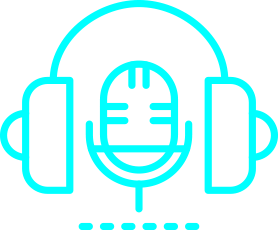
Meet Our Contributors
Sources
- How Much Data Do We Create Everyday? The Mind-Blowing Stats Everyone Should Read, Forbes
- The Digitization of the World from Edge to Core, IDC
- LinkedIn Workforce Report 2018, LinkedIn
- What is Data Strategy?, Dataversity
- Why Every Business Needs a Data and Analytics Strategy, Bernard Marr & Co.
- Making Advanced Analytics Work for You, Harvard Business Review
- Netflix Quarterly Earnings Report, Q1 2019, Netflix
- Why Every Company Needs A Data Strategy For 2019, Fortune
- The 5 Essential Components of a Data Strategy, SAS
- How Netflix Uses Big Data to Drive Success, Predictive Analytics World