Improve data quality—and inspire confidence
January 28, 2016 | By Lisa Dare, TEKsystems Digital Content Strategist
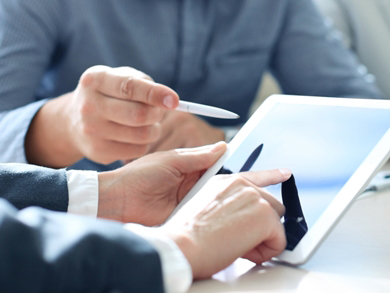
That uncomfortable, uncertain feeling you get when you think about your company’s data? You’re not alone, says James Smith, a practice architect with TEKsystems. “Most companies have no idea about the quality level of their data. They usually find out the hard way after they embark on a new project and have to go back for a lot of cleansing,” says Smith.
Particularly before embarking on a major initiative like implementing BI or migrating data, you need to be very confident in the integrity and security of your data.
“There’s not a hard metric for accuracy but it needs to be clean to a point where you can reproduce results every time you run a report with the same constraints,” says Smith.
A strategy to improve data quality
While it’s often overlooked, having a clear strategy for ongoing data quality maintenance is make or break for your business. Bad data is obviously a problem, but simply lacking confidence in your data (whether fairly or not) can also sabotage your BI and related initiatives.
“What people in charge of data initiatives usually fail to take into account is the ongoing process itself: Who will be responsible for identifying problems in the data, for managing and triaging errors, fixing them, classifying?” says Smith.
To counteract these problems and build confidence in your data—and the decisions it drives—your data quality strategy should address:
- Who can see and manipulate the data
- How your data will be updated and cleansed
- How data accuracy will be verified
- Who is responsible for data
- How data will be integrated into master data
Data quality dashboard: A useful tool for many goals
It’s fairly simple to build a data quality strategy using a visualization tool like Qlikview or Tableau, and it’s well worth doing. Why?
1. To show the quality and validity of your data
If your users have no confidence in the data, you won’t get new requests for analysis and projects. But if your data is reliable and dependable—and users have confidence that it is—you’ll get a stream of requests for improvements. In fact, new user requests are a good indicator of the health and confidence in your data, says Smith.
2. To show progress in cleansing and managing data
By measuring against a set of quality metrics and socializing results, organizations can track progress and encourage good behavior.
3. To show how the data is used, i.e., those user requests
If you want to get decision-makers to invest in data quality and data tools, it’s critical that you show that real users interact with the data. This also helps you figure out what analysis tools will be useful investments for your organization.
Data cleansing tools: Effective use and limitations
You should invest in a data cleansing tool, which can take care of perhaps 80 percent of data inconsistencies by automating cleanup or setting up data entry fields to have limited variation, e.g., placing number limits on zip code fields or even checking the codes against a database for accuracy. Dimitry Borochin, a data services architect with TEKsystems, explains how it works. “As data gets loaded into your databases or is already at rest, it triggers a process to validate data against a knowledge base of rules. Fields and records that don’t pass the validation rules get automatically corrected using ‘action rules’ or flagged for manual review by subject matter experts, as in data stewards.”
Organizations that hum along without measuring and monitoring may keep the lights on, but there is little opportunity for progress or conscious, informed decision making.
That means you need human data stewards who maintain and augment that knowledge base over time, drawing on their understanding of the business and downstream ramifications of rules, explains Borochin. Data steward isn’t a full-time role, but is typically a business stakeholder. In addition to maintaining rules, the steward should advocate for data governance and best practices in his or her unit.
For instance, if your sales team relies on a CRM like Salesforce, the data steward periodically reviews the data (often quarterly) to discover patterns and outliers in data entries and thinks about what they mean for your organization. He or she may find overlapping naming conventions for your lines of business that make for segmented data and require a lot of time in manual integration—which isn’t just wasted time, but allows a lot of results variation to creep in.
Who’s responsible for data?
Data should be the responsibility of every person in your organization. Period. “Conscious use of data is the difference between existing and progressing,” says Borochin. “Data is like the human brain. Your brain powers involuntary functions like keeping your heart beating but it’s conscious thought that makes you grow and learn and determine your own fate. Organizations that hum along without measuring and monitoring may keep the lights on, but there is little opportunity for progress or conscious, informed decision making.”
Here are the key players to maintaining and advocating for data integrity:
Data experts: You’ll need data architects who understand not just the technical concepts like data warehousing, but what they mean for your organization. For instance, how much is fast performance and a future-proofed data architecture worth? The right balance depends on knowing the business well.
Data governance board:
A data board is critical for:
1. Managing data system rules
2. Creating and communicating data standards to users
3. Advocating for data cleansing and integration projects
4. Determining data access levels, i.e., permissions
Data scientists (and the role of data lakes): There’s a lot of controversy over what this job title means, the qualifications for it and whether granting a data scientist access to your data is a good idea. Smith thinks you should have data scientists, saying, “Data isn’t meant to be a black box that only a few can use; however, you also can’t turn data loose to the wrong people who might either use it for wrongdoing or aren’t knowledgeable about how to analyze it and misconstrue it. And while you can’t fully prevent that, proper data governance means knowing who is using data and for what purpose.”
Some organizations use the term loosely, looking for someone who isn’t a developer, but has advanced skill in data analysis and reporting best practices, who knows enough to understand what a threshold is.
Other organizations need a higher-skilled person who can use artificial intelligence methods to create new kinds of data analysis and predictive analytics. This person builds models to determine probabilities to certain outcomes based on historical data. “A real data scientist is more than an analyst who queries data in interesting ways. He or she needs the ability to explore as many features and attributes in the data as possible. Ideally, they should have access to a data lake,” says Borochin.
How does a data lake provide self-service and flexibility for data scientists? It’s a single platform where loosely related, structured and/or unstructured data co-exist. By hosting this data in a single place and providing an array of tools to structure, relate and transform it, you allow data scientists the access they need to build data sets with a rich set of attributes.
Another benefit of having a data lake beyond providing a staging condition (data talk for initial loading), is that it offers a higher degree of reliability and accuracy in data cleansing routines.
Focus on what matters
Don’t stretch your organization thin chasing every new technology or tool—you need a strong data quality program as the foundation for strong data outcomes. A data platform you invest in should be flexible, extensible and responsive to business needs. Build a good foundation, look at features that support and enhance business processes and strategic initiatives, and consciously adopt a culture of experimentation.
You believe in the transformative power of data
Awaken your data.