AI and automation begin with data
Why a strong data strategy is needed to successfully operationalize AI
Aug. 10, 2020 | By: Ramesh Vishwanathan
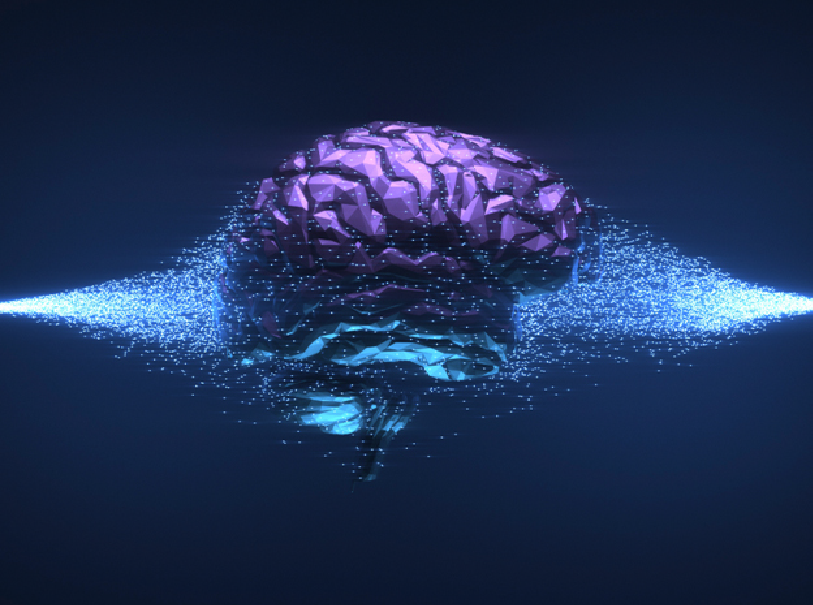
Artificial intelligence, machine learning, automation. The future is now. The benefits of AI have arrived—from new ways of working to putting data to work. Organizations are trailblazing into cloud automation, ready to activate speed, efficiency and cost savings. As an additional layer, AI is now being thrown into the mix to take data analytics efforts from being a tool to analyze causality post events, to being an autonomous instrument that can make decisions in real time to avoid those events from even taking place. But automating cloud infrastructures requires comprehensive ideation and execution—beginning with the data itself.
A dynamic duo: Cloud automation and AI
Automation and AI go hand in hand. Think of automation as the arms and legs, while AI is the brain. To have any meaningful impact, both need to work together to produce results.
Automating cloud infrastructures enables you to scale at speed, with many ways and tools to help do so. Automation needs a continuous feedback and correction loop as guardrails to ensure it doesn’t drive you down a cliff. Traditionally, rule-based alerts and monitoring systems were leveraged as mechanisms to take control of exceptions. With the scale of systems growing exponentially, rule-based alerts have produced more “noise” rather than providing actionable insights. It’s no longer a viable option to manage systems based on rules or to have human intervention for all the alerts and events being generated. Leveraging AI within cloud-based infrastructures provides much-needed rails that can be leveraged to reap the benefits of automation. AI-driven operation automations can reduce long-term costs by offloading repetitive, manual decisions around provisioning, configuration and capacity. Given the right set of data, AI-driven operations enable organizations to move toward proactive management at speed from a traditionally reactive approach to IT operations. Keep this in mind: the predictive power of AI is only as good as the strength and health of your data.
Accurate data fuels…everything
While AI can organize the way your data is consumed and updated, automation still works within the confines of what you feed it. One of the most valuable steps you can take to ensure your automation models are successful is validating the quality of your data.
Any autonomous engine is only as good as the quality and rigor of training it was provided. Inherited data issues will invalidate the process and potentially have negative impacts on business value. To power business decisions with predictive analytics, processing and creating a meaningful and clear data strategy will make all the difference.
Bridging the gap between AI and data science
Collecting and using data is at the core of automation and AI, but to fully take advantage of it, the process of digitizing data—data acquisition—through data science is needed.
Data science has been in existence for decades, and two of the biggest challenges that organizations have always faced in putting their data to work is:
- Integrating and operationalizing AI into legacy processes that don’t necessarily lend themselves to automation
- Acquisition and management for quality data
Organizations that have set the stage with digitization and business transformation provide much needed thrust in capturing digitized data, as well as lays the foundation for bringing in automation. Cloud infrastructure is one such transformation that provides the right platform for helping organizations adopt AI and automation to scale up their IT operations. Just the existence of your collected data is not enough. To truly realize its value, you need to connect and streamline data science to extract ideas and solutions from your insights—ultimately synergizing the efforts between data and any process automation. But even then, it’s challenging to align AI to the business and integrate it with strategic applications and processes.
To operationalize AI, you need a holistic approach that focuses on the complete life cycle of AI and aligns automation to enterprise processes. It comes full circle—a strong data strategy will help your automation strategy, which, in turn, will lend itself to manage data quality, data protection and database storage efficiencies.
Strengthening your data strategy and ensuring you’re capturing accurate data can give organizations better command of their data, drawing real-time insights. By automating your cloud strategy and leveraging predictive data analytics, you can react to your organization needs at speed and be prepared to handle the agile needs of the competitive market space.
Ramesh Vishwanathan is a data insights leader, innovator and AI evangelist. He leads TEKsystems’ data insights practice, focusing on cloud, big data and AI strategy. He is an expert at helping customers adopt and mature AI and helps lead them to data-driven automation and intelligence-driven business processes.