Insights
Version Next, Now
Advancing Business Solutions with Autonomous AI Agents
In this issue we explore how agentic AI is redefining what’s possible across the enterprise—from transforming customer experiences to accelerating innovation and operational efficiency.
State of Digital Transformation Report
Leveraging Momentum
Discover how leading organizations strategically stand out from the laggards and learn actionable insights to propel your company forward in 2025.
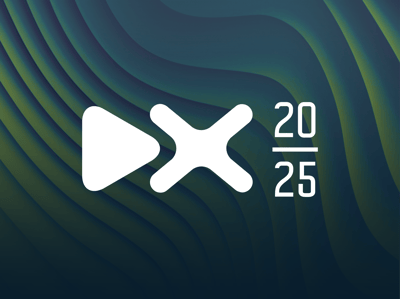