Paperless Precision
VERSION NEXT, NOW
APAC EDITION
Unleashing NLP in Business Management
The Change Agent
In an era where efficiency and accuracy are paramount, businesses are constantly seeking ways to streamline operations and reduce manual workloads. Increase productivity and revolutionise modern business operations with natural language processing (NLP) and generative AI.
A New Era in Document Management
Imagine a world where mountains of paperwork are handled effortlessly, where contracts, reports and emails are meticulously organised and understood in mere seconds. In this world of advanced document management and generative AI, businesses operate with unprecedented efficiency thanks to the seamless integration of cutting-edge technology. This digital transformation is not the stuff of science fiction, but a present-day reality made possible by the advancements in natural language processing (NLP).
NLP, a subfield of artificial intelligence, focuses on the interaction between computers and human language. By leveraging sophisticated algorithms and vast amounts of data, NLP enables machines to comprehend, interpret and generate human language in a way that is meaningful and useful.
From automating customer service responses to analysing vast troves of legal documents, natural language processing use cases, enhanced by generative AI, are revolutionising business operations across various industries, making tasks that once seemed insurmountable now manageable with the click of a button.
As we delve deeper into the NLP applications and capabilities, we uncover the true potential of this transformative technology. Traditionally, document management used to involve time-consuming manual processes that were prone to human error. Employees had to sort, categorise and file documents by hand, often leading to inefficiencies and bottlenecks. Searching for specific information within these documents was akin to finding a needle in a haystack, and the physical storage of paper documents required considerable space and resources.
In contrast, modern Intelligent Document Processing (IDP) solutions, powered by NLP and enhanced by generative AI, have redefined document management for businesses. IDP automates the extraction, classification and validation of information from a variety of document types, both structured and unstructured. These systems can swiftly process invoices, contracts and forms, accurately pulling relevant data and integrating it into digital workflows.
The shift from traditional methods to IDP solutions exemplifies the profound impact of technology on modern business operations. By adopting IDP, organisations can streamline their workflows, reduce operational costs and allocate human resources to more strategic tasks, ultimately driving productivity and innovation.
How Does NLP Work?
NLP works by combining computational linguistics with machine learning and deep learning techniques. It is a transformative technology that enhances the ability of machines to understand and interact with human language. Its integration into Intelligent Document Processing significantly improves efficiency and accuracy in handling large volumes of documents, enabling businesses to automate and streamline various processes.
The process typically involves several key steps:
1. Text Preprocessing
- Tokenization: splitting text into words, phrases or symbols
- Normalisation: converting text to a standard format (e.g., lowercasing, removing punctuation)
- Stop words removal: removing common words that may not be useful for analysis (e.g., “and” or “the”)
- Stemming and lemmatization: reducing words to their root forms
2. Feature Extraction
- Bag of words: representing text by the frequency of each word
- TF-IDF (term frequency-inverse document frequency): weighing the importance of a word in a document relative to a collection of documents
- Word embeddings: representing words in continuous vector space (e.g., Word2Vec, GloVE)
3. Model Training
- Supervised learning: training models on labeled data for tasks like sentiment analysis or named entity recognition
- Unsupervised learning: extracting patterns from unlabeled data, such as topic modeling
4. Evaluation
- Assessing model performance using metrics like accuracy, precision, recall and F1 score
5. Deployment
- Implementing the model in applications such as chatbots, search engines and recommendation systems
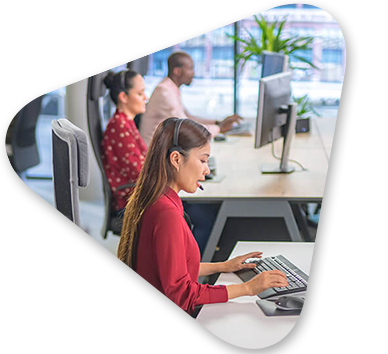
Organisations must think about how to combine the best of both worlds, providing specific information about guidelines and expectations for a particular domain or industry rather than just generic responses. This is where NLP, fueled by Gen AI, becomes a differentiator.
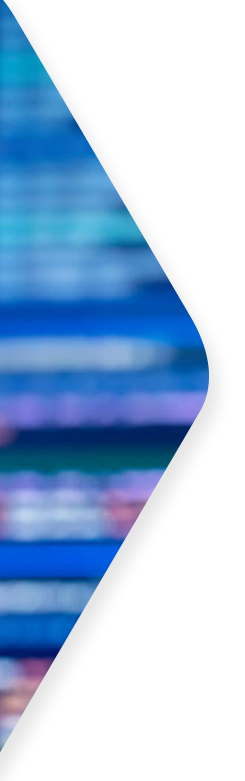
Our Perspective
By integrating NLP into IDP solutions, organisations achieve higher accuracy, efficiency and scalability in document processing, leading to significant cost savings, improved compliance and better decision-making.
The global NLP market is projected to increase from $36.42 billion in 2024 to $156.80 billion by 2030, reflecting a compound annual growth rate (CAGR) of 27.5%.1
Industries Most In-Demand for NLP are Financial Services and Healthcare
Enhanced search capabilities, driven by contextual and semantic search, allow users to perform more intuitive and accurate searches, particularly useful in domains like legal, medical and technical documentation. NLP improves data normalisation and standardisation, ensuring consistent formatting and enriching extracted data by linking related information.
NLP enhances compliance and risk management by identifying and extracting information required for compliance reporting, auditing documents for standards, and flagging noncompliant content. It automates end-to-end document processing workflows, integrating with systems like ERP, CRM or RPA platforms, reducing manual intervention and increasing efficiency. Intelligent routing capabilities direct processed documents based on content and context.
Lastly, NLP enhances user experience by providing intuitive interfaces for interacting with document processing systems through natural language commands and queries, making systems more user-friendly and accessible.
The convergence of NLP with IDP uses advanced technologies to automate the extraction, interpretation and processing of data from various document types across an organisation. Generative AI enhances these capabilities further by interpreting complex documents such as doctors notes and technical diagrams, providing summaries and insights that older AI models couldn’t achieve.
What changed since generative AI came into play is that generative AI has been trained on how humans communicate, and that training was not done like the old deterministic training methods, giving NLP responses more humanist qualities.
Challenges of NLP-Driven IDP
NLP faces challenges like language differences, data training, ambiguities, misspellings, bias and phrases with multiple meanings. These challenges impact its effectiveness and efficiency.
Languages vary in syntax, grammar and semantics, making it difficult for NLP systems to perform well across multiple languages. Training NLP models requires large volumes of high-quality, annotated data, which is resource-intensive and time-consuming. The quality of training data directly impacts model performance, with inaccuracies or biases leading to poor results.
Developing NLP systems involves complex processes requiring expertise and computational resources. Rapid advancements in NLP mean keeping up with the latest techniques can be challenging. Natural language is inherently ambiguous, with words and sentences often having multiple interpretations, requiring sophisticated contextual understanding.
Misspellings and typographical errors in real-world text data challenge NLP models’ accuracy. Bias in NLP models, learned from training data, can lead to unfair outcomes, requiring careful selection and preprocessing of training data. Many words and phrases have multiple meanings depending on context, necessitating large amounts of contextual data and sophisticated modeling techniques for effective disambiguation.
Addressing these challenges requires ongoing research, advanced techniques and comprehensive strategies to ensure NLP systems are accurate, fair and robust.
Benefits of NLP-Driven IDP
NLP offers numerous benefits in business operations, customer interactions and data analysis. One primary benefit is enhanced customer service. NLP-powered chatbots and virtual assistants provide 24/7 support, handling routine queries and freeing human agents for complex issues, resulting in faster response times and higher customer satisfaction. Sentiment analysis tools monitor customer feedback in real time, allowing prompt issue resolution.
NLP improves efficiency and automation by handling repetitive tasks like data entry, document processing and information extraction, minimising errors and increasing operational efficiency. In finance, it automates information extraction from financial documents, speeding up loan approvals and compliance checks. In the legal field, NLP streamlines contract analysis and legal research.
NLP enhances data analysis by extracting insights from unstructured text data, informing strategic decisions, improving marketing campaigns and providing deeper customer understanding. It helps organisations maintain a competitive edge by monitoring and analysing competitor activities and market trends.
NLP contributes to better decision-making and knowledge management by improving search result accuracy, aiding quick information retrieval and enhancing productivity. Intelligent document processing solutions categorise and tag documents, making retrieval efficient. This is beneficial in fields like healthcare, improving access to relevant medical literature and patient records.
Despite challenges, implementing generative AI and NLP in IDP solutions offers significant benefits, including higher accuracy, efficiency, and scalability, leading to cost savings, improved compliance, and better decision-making.
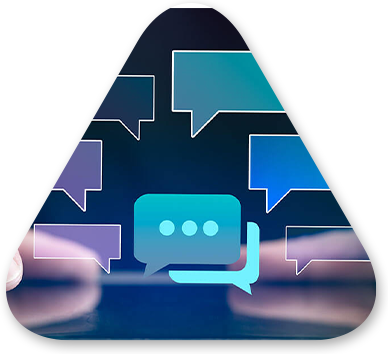